Chi Square Analysis - The Open University
www open ac uk/socialsciences/spsstutorial/files/tutorials/chi-square pdf
the same as the expected frequencies (except for chance variation) observed frequency-distribution to a theoretical expected frequency-distribution
SPSS: Expected frequencies, chi-squared test In-depth example
www sfu ca/~jackd/Stat203_2011/Wk12_2_Full pdf
Most important things to know: - How to get the expected frequency from a particular cell - Chi-squared is a measure of how far the observed frequencies are
Chi-Square
www d umn edu/~rlloyd/MySite/Stats/Ch 2013 pdf
Step 1: Arrange data into a frequency/contingency table Step 2: Compute Expected Frequencies Based Upon Null Hypothesis
?2 Test for Frequencies
courses washington edu/psy315/tutorials/chi_2_test_frequencies_tutorial pdf
17 jan 2021 Like all statistical tests, the ?2test involves calculating a statistic that measures how far our observations are from those expected under the
2 X 2 Contingency Chi-square
web pdx edu/~newsomj/uvclass/ho_chisq pdf
examine the expected vs the observed frequencies The computation is quite similar, except that the estimate of the expected frequency is a little harder
Chi-Square Tests and the F-Distribution Goodness of Fit
www3 govst edu/kriordan/files/mvcc/math139/ pdf /lfstat3e_ppt_10 pdf
To calculate the test statistic for the chi-square goodness-of-fit test, the observed frequencies and the expected frequencies are used The observed frequency
1 4 Chi-squared goodness of fit test 1 Introduction 2 Example
www lboro ac uk/media/media/schoolanddepartments/mlsc/downloads/1_4_gofit pdf
estimated from the (sample) data used to generate the hypothesised distribution From these we can calculate the expected frequencies
Chi-Squared Tests
www thphys nuim ie/Notes/EE304/Notes/LEC14/ChiSlide pdf
If the 6-sided die is fair, then the expected frequency is on the null hypothesis and then compare the expected frequencies with the actual frequencies
Week 6: Frequency data and proportions - UBC Zoology
www zoology ubc ca/~whitlock/bio300/labs/LabManual/Week 2006 20-- 20FREQUENCY 20DATA pdf
categorical variable to the frequencies predicted by a null hypothesis than 25 of the expected frequencies are less than 5 and none is less than 1 )
Ex 8- Chi-squared Mapping Exercise pdf - webspace ship edu
webspace ship edu/pgmarr/Geo532/Ex 208- 20Chi-squared 20Mapping 20Exercise pdf
difference between the observed and expected frequencies ij is the expected frequency, R is the row, C is the column, and n total observations
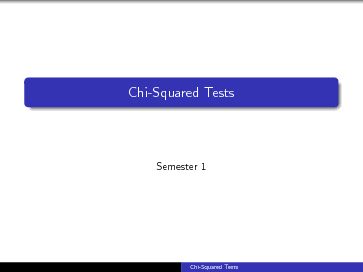
100438_3ChiSlide.pdf
Chi-Squared Tests
Semester 1
Chi-Squared Tests
Goodness of Fit
Up to now, we have tested hypotheses concerning the values of population parameters such as the population mean or proportion.We have not considered testing hypotheses about the form of a population's distribution.We next consider the problem of determining whether or not a population follows a particular distribution.
Chi-Squared Tests
Goodness of Fit
For example, we may be interested in determining whether the number of emails arriving per minute at a server follows a Poisson distribution or not.Similarly, we may wish to test if the lengths of components
from an automated process follow a normal distribution.Another similar question is whether a 6-sided die is fair or not.
Chi-Squared Tests
Goodness of Fit
The general procedure for testing hypotheses on the distribution of a population is as follows. (i) The null hyp othesisH0is that some distribution describes the population.(ii)W echo osea sample of siz enfrom the population. This might involve recording the results ofnrolls of the die or recording
the number of mails arriving innminute-long intervals.(iii)The observations in our sample a regroup edinto k\bins" or
\classes" and we record the number of observations that fall into each bin or thefrequencyof each bin.Oirepresents the
number of observations orobserved frequencyfor theithbin.(iv)Under the null hyp othesis,w ecan calculate the expected
frequency E ifor each bin.Chi-Squared Tests
Goodness of Fit
(v)
The test statisticwe use is
20=kX i=1(Ei Oi)2E i: If the null hypothesis is true, then20has approximately a chi-squared distribution withk p 1 degrees of freedom. Herepis the number of parameters of the distribution that
we have to estimate with our sample data.(vi)W ereject H0at the signicance levelif the value of20calculated with our sample data exceeds the critical value
2k p 1;which we obtain from a table of chi-square critical
values.
Chi-Squared Tests
Goodness of Fit
For example:
For testing the fairness of a die, we would use 6 bins for the numbers 1 to 6 andOiwould count the number of times the numbericame up in thenrolls.For the email server example, the bins might represent 0 emails, 1 email, 2 emails, 3 emails and4 emails. In this case, we would have 5 bins in total, and O
1would count the number of minutes in which we received 0
emails, O
2the number of minutes in which we received 1 email,
O
3the number of minutes in which we received 2 emails and
so on.
Chi-Squared Tests
Goodness of Fit
If the 6-sided die is fair, then the expected frequency is E i=n6 for each bin.If the number of emails arriving at the server per minute follows a Poisson distribution with mean, the expected number of minutes in which no emails arrive would be e n: The expected number of minutes in which 1 email arrives would bee 11! n and so on.
Chi-Squared Tests
Goodness of Fit
The key point is that we can compute expected frequencies based on the null hypothesis and then compare the expected frequencies with the actual frequencies observed in a sample. When the deviation between the expected frequencies and the observed frequencies istoo largewe reject the null hypothesis concerning the population.To determine when the dierence between observed and expected frequencies is too large, we use a special distribution known as the chi-squared di stribution.
Chi-Squared Tests
Poisson Goodness of Fit
Example
The number of emails arriving at a server per minute is claimed to follow a Poisson distribution. To test this claim, the number of emails arriving in 70 randomly chosen 1-minute intervals is recorded. The table below summarises the results.
Number of emails01234Frequency132223120
Test the hypothesis that the number of emails per minute follows a Poisson distribution? Use a signicance level of= 0:05.Chi-Squared Tests
Poisson Goodness of Fit
To calculate the expected frequencies, we need the Poisson parameter. This is simply the mean number of emails per minute. We need to estimate this from the sample data: =13(0) + 22(1) + 23(2) + 12(3)70 = 1:49:Our Null Hypothesis isH0: Number of emails per minute has a Poisson Distribution with= 1:49.H
1: Number of emails per minute does not have a a Poisson
Distribution with= 1:49.Signicance Level:= 0:05.Test Statistic: We treat the last two bins as one (as no
minutes contained 4 or more calls) so the number of bins is k= 4. 20=4X i=1(Ei Oi)2E i:
Chi-Squared Tests
Poisson Goodness of Fit
We rejectH0if our sample data gives a value of
20> 22;0:05= 5:99. We have lost two degrees of freedom
because we have to estimate the parameterfrom sample data.To do the calculation, we require:
Number of emailsObserved Freq.Expected Freq.
01315.78
12223.51
22317.5
31213.2
Chi-Squared Tests
Poisson Goodness of Fit
The actual value of20is then:
(15:78 13)215:78+(23:51 22)223:51+(17:51 23)217:51+(13:2 12)213:2 which is equal to 2.417.We cannot reject the null hypothesis at the 5% level of signicance.
Chi-Squared Tests
Binomial Goodness of Fit
It is also possible to perform a goodness of t test for
distributions other than the Poisson distribution.The approach is essentially the same - all that changes is the
distribution used to calculate the expected frequencies.We next consider an example based on the Binomial
distribution.
Chi-Squared Tests
Binomial Goodness of Fit
Example
Bits are sent over a communications channel in packets of 8. In order to characterise the performance of this channel, 80 packets are sent over the channel and the number of corrupted bits in each packet is recorded. The results of this experiment are recorded below. Number of Corrupt Bits01234Number of Packets35311040 Test the hypothesis that the number of corrupted bits in a packet sent over this channel follows a binomial distribution. Use a signicance level of= 0:025Chi-Squared Tests
Binomial Goodness of Fit
To calculate the expected frequencies, we need the binomial parameterp.We need to estimate this from the sample data. Out of the 640 bits sent over the channel, 63 were corrupt.
So our estimate ofpis63640
= 0:098Chi-Squared Tests
Binomial Goodness of Fit
H
0: Population is binomial withp= 0:098.H
1: Population is not binomial.Signicance Level:= 0:025.Test Statistic: We treat the last two bins as one (as no
packets contain 4 or more corrupt bits) so the number of bins isk= 4. 20=4X i=1(Ei Oi)2E i:We rejectH0if our sample data gives a value of
20> 22;0:025= 7:378. We have lost two degrees of freedom
because we have to estimate the parameterpfrom sample data.
Chi-Squared Tests
Binomial Goodness of Fit
To do the calculation, we require:
Number of Corrupt BitsObserved Freq.Expected Freq.
03535.04
13130.48
21011.6
342.88
The actual value of20is then:
(35:04 35)235:04+(30:48 31)230:48+(11:6 10)211:6+(2:88 4)22:88= 0:665:We cannot reject the null hypothesis at the 1% level of
signicance.
Chi-Squared Tests
Normal Goodness of Fit
The nal example of goodness of t that we shall consider is for the Normal distribution.For this case, the situation is a little more complicated as the distribution is continuous. This means that we need to be more careful in selecting the bins.In practice, it is usual to choose bins so that the expected frequency for each bin is the same.We shall see how to do this in an example below.
Chi-Squared Tests
Normal Goodness of Fit
Example
A text processing tool can be downloaded from a particular webserver. The administrator of the server wishes to test if the download times are adequately described by a normal distribution. A random sample of 80 users is selected and their download times recorded. The mean and standard deviation of the download times (in seconds) for the sample are 20.2 and 2.1 respectively.
Chi-Squared Tests
Normal Goodness of Fit
Suppose we wish to use 8 bins.
We rst nd the intervals that divide the standard normal distribution into 8 equal parts.From the table of standard normal probabilities we can see that these intervals are: ( 1; 1:15];( 1:15; 0:675];( 0:675; 0:32];( 0:32;0]
and their mirror images on the other side of 0.This allows us to construct the bins in which to group our
data.
Chi-Squared Tests
Normal Goodness of Fit
The rst bin will bex20:2 1:15(2:1) = 17:785, the second bin will be 17:785