A geometric random variable with parameter p will be denoted by GE(p), and it has the probability mass function (PMF); p(1 ? p)n?1, for n = 1,2, Now we
Another characterization based on the quadratic statistics X 2 and (X + a) 2 for some constant a r 0 will be given In Section 3, the decomposition of a larger
Some key words: Bivariate distribution; Multivariate normal distribution; conditioning mechanism can be used to obtain the skew-normal distribution as a
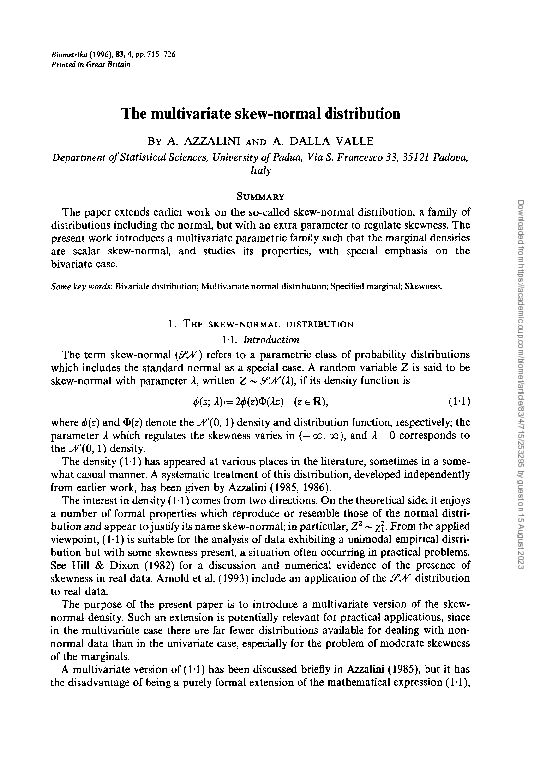
41134_683_4_715.pdf
Biometrika (1996), 83, 4, pp. 715-726
Printed
in Great Britain Th e multivariat e skew-norma l distributio n B Y A . AZZALIN I AN D A . DALL A VALL E
Department
of Statistical Sciences, University of Padua, Via S. Francesco 33,
35121
Padova, Italy
SUMMAR
Y Th e pape r extend s earlie r wor k o n th e so-calle d skew-norma l distribution , a famil y o f distribution s includin g th e normal , bu t wit h a n extr a paramete r t o regulat e skewness . Th e presen t wor k introduce s a multivariat e parametri c famil y suc h tha t th e margina l densitie s ar e scala r skew-normal , an d studie s it s properties , wit h specia l emphasi s o n th e bivariat e case . Some key words: Bivariat e distribution ; Multivariat e norma l distribution ; Specifie d marginal ; Skewness . 1 . TH E SKEW-NORMA L DISTRIBUTIO N 11 . Introduction Th e ter m skew-norma l (SfJf} refer s t o a parametri c clas s o f probabilit y distribution s whic h include s th e standar d norma l a s a specia l case . A rando m variabl e Z i s sai d t o b e skew-norma l wit h paramete r X, writte n Z ~ SfJ^iX), i f it s densit y functio n i s
0(z;A).
= ty(z)
. Th e interes t i n densit y (11 ) come s fro m tw o directions . O n th e theoretica l side , i t enjoy s a numbe r o f forma l propertie s whic h reproduc e o r resembl e thos e o f th e norma l distri - butio n an d appea r t o justif y it s nam e skew-normal ; i n particular , Z 2 ~ Xi - Fro m th e applie d viewpoint , (1-1 ) i s suitabl e fo r th e analysi s o f dat a exhibitin g a unimoda l empirica l distri - butio n but wit h som e skewnes s present , a situatio n ofte n occurrin g i n practica l problems . Se e Hil l & Dixo n (1982 ) fo r a discussio n an d numerica l evidenc e o f th e presenc e o f skewnes s i n rea l data . Arnol d e t al . (1993 ) includ e a n applicatio n o f th e VJ/" distributio n t o rea l data . Th e purpos e o f th e presen t pape r i s t o introduc e a multivariat e versio n o f th e skew - norma l density . Suc h a n extensio n i s potentiall y relevan t fo r practica l applications , sinc e i n th e multivariat e cas e ther e ar e fa r fewe r distribution s availabl e fo r dealin g wit h non - norma l dat a tha n i n th e univariat e case , especiall y fo r th e proble m o f moderat e skewnes s o f th e marginals . A multivariat e versio n o f (11 ) ha s bee n discusse d briefl y i n Azzalin i (1985) , bu t i t ha s th e disadvantag e o f bein g a purel y forma l extensio n o f th e mathematica l expressio n (11) ,Downloaded from https://academic.oup.com/biomet/article/83/4/715/253295 by guest on 15 August 2023
716 A. AZZALINI AND A. DALLA VALLE
an d it s marginal s d o no t enjo y th e propert y o f bein g skew-norma l densities , a s remarke d b y An a Quirog a i n he r 199
2 doctora l dissertatio n a t th e Universit y o f Uppsala . T o achiev e a multivariat e distributio n wit h specifie d marginals , a standar d metho d i s t o us e th e Farlie-Gumbel-Morgenster n formula , whic h i n th e bivariat e cas e take s th e for m T7 ( v Y _ 1 - / 7 I Y \J? (Y ^ [ " 1 -i- ni 1 J* (Y W i 1 V (~Y W~\V 1 > 2/ - 1 \*^1 / 2\ 2/ L ' X 1 \ 1)) X 2\ 2//J> wher e F x an d F 2 denot e th e margina l distribution s an d a i s a paramete r varyin g i n ( - 1,1) . On e limitatio n o f thi s approac h i s tha t agai n th e extensio n fro m th e univariat e t o th e bivariat e cas e i s o f purel y forma l nature , an d i t i s no t linke d t o th e intrinsi c propertie s o f th e Fj's. I n particular , th e abov e formul a doe s not produc e th e bivariat e norma l distri - butio n whe n F 1 (x ) = F 2 (x ) = O(x) . Anothe r disadvantag e i s tha t th e rang e o f achievabl e correlation s obtaine d b y varyin g a i s restricte d t o th e interva l ( - 5,3 ) (Schucany , Par r & Boyer , 1978)
. I n th e presen t paper , w e conside r a differen t approach , mor e directl y linke d t o th e natur e o f th e scala r ifjf distribution , whic h ensure s tha t th e marginal s ar e scala r SfJf variates . Afte r presentin g th e genera l /c-dimensiona l version , w e focu s o n th e bivariat e case , includin g a n illustratio n wit h rea l data . 1-2 . Some formal preliminaries Befor e tacklin g th e multivariat e case , i t i s usefu l t o recal l som e propertie s o f th e scala r 'Jf distribution , fo r late r use . Th e momen t generatin g functio n an d th e first moment s o f Z ar e give n i n Azzalin i (1985) . I n particular ,
E(Z)=(-)
d, var(Z)=l--<5 2 , (1-2 ) wher e d i s relate d t o X vi a th e relationship s X Also , th e inde x o f skewness , varie s i n th e interva l ( - 0-995,0-995) . Henz e (1986 ) ha s give n th e genera l expressio n o f th e od d moment s i n close d form ; th e eve n moment s coincid e wit h th e norma l ones , becaus e Z 2 ~ x\-
PROPOSITIO
N X.lfY and W are independent JV{0, 1 ) variates, and Z is set equal to Y conditionally on XY>W, for some real X, then Z ~ SfJ/~{X). Thi s resul t i s essentiall y equivalen t t o th e proo f tha t (11 ) i s a densit y function , give n i n Azzalin i (1985) . Fo r rando m numbe r generation , i t i s mor e efficien t t o us e a varian t o f thi s result , namel y t o pu t XY>W, Y ifXY^W, henc e avoidin g rejectio n o f samples
.= {YDownloaded from https://academic.oup.com/biomet/article/83/4/715/253295 by guest on 15 August 2023
Multivariate skew-normal distribution 717
Th e nex t resul t follow s immediatel y fro m Propositio n 1 o n settin g X =
PROPOSITIO
N 2 . If(X, Y) is a bivariate normal random variable with standardised mar- ginals and correlation 5, then the conditional distribution of Y given X >0 is SfJf{X(b)}. Thi s representatio n o f th e SfJf distribution , derive d i n a differen t wa y b y Arnol d e t al . (1993) , i s interestin g sinc e i t link s th e Sfjf distributio n t o a censorin g operatio n o n norma l variates , a situatio n naturall y occurrin g i n a larg e numbe r o f practica l cases . A simila r conditionin g mechanis m ca n b e use d t o obtai n th e skew-norma l distributio n a s a prio r distributio n fo r th e mea n o f a norma l variable , i n a Bayesia n framewor k (O'Haga n &
Leonard
, 1976)
. Th e followin g furthe r representatio n o f Z , include d i n Azzalin i (1986) , i s familia r i n th e econometri c literatur e o n th e stochasti c frontie r model , startin g fro m Aigner , Lovel l &
Schmid
t (1977) . Andel , Netuk a & Zvar a (1984 ) obtai n (11 ) a s th e stationar y distributio n o f a nonlinea r autoregressiv e process , wit h transitio n la w relate d t o th e nex t result .
PROPOSITIO
N 3. If Y O and Y t are independent ^V(0, 1) variables and d e ( - 1,1) , then is 2 . A MULTIVARIAT E EXTENSIO N 21
. Transformation method Fo r th e fe-dimensional extensio n o f (11) , w e conside r a multivariat e variabl e Z suc h tha t eac h componen t i s skew-normal . I t i s the n natura l t o defin e th e join t distributio n o f Z a s bein g multivariat e yjf.
Conside
r a /c-dimensiona l norma l rando m variabl e Y={Y U ... , Y k ) T wit h standardise d marginals , independen t o f Y o ~ J/~(Q, 1) ; thu s 0 wher e ¥ i s a k x k correlatio n matrix . I f (<5 1; ... , 3 k ) ar e i n ( - 1,1) , defin e [j=l,...,k), (2-2 ) s o tha t Zj~£f^V{X(5 ] )}, b y Propositio n 3 . Computatio n o f th e distributio n o f Z = (Z X) ... , Z k ) r i s trivia l bu t lengthy ; th e basi c step s ar e reporte d i n th e Appendix . Th e final expressio n fo r it s densit y functio n i s / t (z ) = 2^(z;fl)
718 A. AZZALINI AND A. DALLA VALLE an d k (z; Q ) denote s th e densit y functio n o f th e fc-dimensional multivariat e nonna l distri - butio n wit h standardise d marginal s an d correlatio n matri x Q . W e shal l sa y tha t a rando m variabl e Z wit h densit y functio n (2-3 ) i s a fc-dimensional skew-norma l variable , wit h vecto r X o f shap e parameter s an d dependenc e paramete r W. Fo r brevity , w e shal l writ e z ~ yjr^k,^)- (2-7 ) 22
. Conditioning method A n immediat e questio n i s whethe r w e woul d obtai n th e sam e clas s o f densit y function s i f w e star t fro m anothe r propert y o f th e scala r SfJf distribution , instea d o f Propositio n 3 . Propositio
n 2 suggest s th e followin g scheme . Le t X = (X o , X lt ..., X k ) T b e a (k + 1) - dimensiona l multivariat e norma l rando m variabl e suc h tha t X ~ jr k + i(y), II ), (^o) wit h standardise d marginal s an d correlatio n matri x / I 5, .. . 5 k \ \s k Iand consider the distribution of (Xu ... ,Xk) given Xo > 0. By Proposition 2, each of these conditional distribution s i s a scala r SfJf, specificall y SfjV{X(dj)}, fo r j = 1,... , k, an d i t woul d b e reasonabl e t o sa y tha t (X u ..., X k ) conditionall y o n J 0 > 0 i s a multivariat e skew-norma l rando m variable . However , i t ca n b e show n tha t th e clas s o f distribution s obtaine d b y thi s conditionin g metho d i s th e sam e a s tha t obtaine d b y th e transformatio n metho d o f §21 . T o prov e this , conside r th e followin g transformatio n o f th e component s o f th e Vs, define d i n (21) : Clearly
, X' = (X' o ,..., X' k ) T i s stil l a multivariat e norma l rando m variabl e wit h standard - ise d marginals , an d th e resultin g correlation s ar e corr(-X"o , X'j) = Sj (j = 1,... , k), con(X h Xj) = co i j = d i d J + \l/ { j(l - df) i (l - dj) i (i>0,j>0), (210 ) wher e \\i ii i s th e correspondin g elemen t o f *F . Therefor e X' ha s th e sam e distributio n a s X introduce d fo r th e conditionin g method . Th e transformatio n metho d an d th e conditionin g metho d diffe r i n tha t th e forme r replace s Y o b y \Y 0 \, whil e th e latte r require s Y o > 0 . Th e symmetr y o f th e ^"(0,1 ) densit y render s thes e tw o operation s equivalent , an d the n th e distributio n o f {X' u ... , X' k ) con - ditionall y o n X Q > 0 i s th e sam e a s fo r (2-2) , provide d tha t th e generi c elemen t a> y o f Q an d th e correspondin g elemen t \\i {i o f * F ar e relate d a s i n (2-10) . Th e requiremen t o f positiv e deflnitenes s o f Q * i n (29 ) impose s som e restriction s o n th e elemen t o f it, onc e th e <5/ s ar e fixed. Thi s i s wh y i n (2-7 ) w e preferre d t o refe r t o *¥ whic h doe s not hav e suc h a restriction . Obviously , i f th e a>,/ s ar e obtaine d vi a (2-10) , th e con - straint s ar e automaticall y satisfied . Thes e aspect s wil l b e considere d i n greate r detai l i n § 3 , fo r th e cas e k = 2.Downloaded from https://academic.oup.com/biomet/article/83/4/715/253295 by guest on 15 August 2023
Multivariate skew-normal distribution 719
23
. Some formal properties I f Z ha s densit y functio n (2-3) , the n it s cumulativ e distributio n functio n i s F k {Zi,..., z Jk ) = pr{Z 1 ^z l5 ... , Z k ^z k } f 'i C Zk f * "= 2 ... k{v; Q)(u) du dvy... dvk J - o o J - o o J - o o fo r z = (z l5 ... , z t ) T e R k , wit h a define d b y (2-4) . The n wher e {Y o , Y 1; ... , 7 t ) T ha s distributio n (2-1 ) an d %=Y 0 -a T Y, s o tha t (y)~^ +1 { a ( 1 -'C r ~^ T )} - (212 )To conclude, the distribution function of the fc-dimensional variable Z ~ S/'J/'k{X XP) can b e obtaine d fro m (211 ) b y computin g th e distributio n functio n o f a (k + l)-dimensiona l norma l variabl e wit h distributio n (212) . Fo r computin g th e momen t generatin g functio n o f Z , w e nee d th e followin g well - know n result . PROPOSITIO
N 4 . / / U ~ ^(0 , ft), then for any scalar u and v 6 K* . Thi s resul t i s usuall y state d fo r a scala r rando m variable ; se e fo r instanc e Zack s (1981 , pp . 53-4)
. Th e presen t formulatio n i s a trivia l extension , sinc e v T U ~ ^"(0 , v r Qv). Computatio
n o f th e momen t generatin g functio n o f Z i s no w immediate : = 2 J RM{t) = 2 exp(tTz)^t(z; Q) = 2 exp{±{t T nt)}El®{a T (U + Qt)} ] (2-13 ) wher e U ~ ^(0, Q). Fo r computin g th e correlatio n matri x o f Z , i t i s bes t t o refe r t o th e transformatio n metho d o f it s generation . Simpl e algebr a lead s t o (2-14 )Downloaded from https://academic.oup.com/biomet/article/83/4/715/253295 by guest on 15 August 2023 720 A. AZZALINI AND A. DALLA VALLE
takin g int o accoun t (12) . Substitutin g (210 ) i n thi s expression , w e ca n als o writ e Pit = TT. " _ 1r ., w , " _ , c ,» , . I n th e scala r case , changin g th e sig n o f a <$OT(>1 ) variabl e produce s a 5OT( - A ) variable . A simila r propert y hold s i n th e multivariat e case . PROPOSITIO
N 5. If Z~ S/'J/' k {k, *¥) and S = diag(s 1? ... , s k ), where s lt . ..,s k is a sequence whose terms are either + 1 or - 1 , then Th e proo f o f thi s statemen t follow s easil y b y applyin g th e SZ transformatio n t o th e Y/s i n (2-2) . Whe n al l s/ s ar e equa l t o - 1 , w e obtai n th e simpl e cas e (215 ) I f th e conditionin g describe d i n § 2- 2 applie s whe n X o < 0 instea d o f X o > 0 , the n th e resultin g distributio n ha s th e 5 an d X vector s o f reverse d sign . Combinin g thi s fac t wit h (2-15) , w e obtai n th e nex t result . PROPOSITIO
N 6 . If X o is a scalar random variable and X is k-dimensional, such that and Z is defined by ifX o >0,Z = 1 - X otherwise, then Z ~ SfjV k {X(5), *?} , where X(5) is given by (13 ) and the entries of *¥ are computed from those of Cl by solving (210) . A corollar y o f thi s fact , whic h generalise s a propert y o f th e scala r skew-norma l distri - bution , i s obtaine d b y noticin g tha t Z T Q -1 Z i s equa l t o X T Cl~ 1 X i n distribution . PROPOSITIO
N 7 . IfZ ~ £Or t (A , »F ) and Q is given by (2-6) , then 3 . TH E BIVARIAT E SKEW-NORMA L DISTRIBUTIO N 31
. Generalities Fo r th e res t o f th e paper , w e shal l concentrat e o n th e bivariat e case . Settin g k = 2 i n (2-3) , th e densit y functio n o f Z = (Z l s Z 2 ) i s fiitu *a ) = 2^ 2 (zi . z 2 ; (uWoCiZ j + 1 5 2 co)}* ' 5 2 - 5^(0 a 2 ={(1 -co2){\ -(02-5l-5j + 2<51 Multivariate skew-normal distribution721
A n alternativ e wa y t o obtai n (31 ) i s t o conside r th e join t distributio n o f (X U X 2 ), component s o f th e vecto r X = (X o , X 1 , X 2 ) T distribute d a s (2-8) , conditione d o n X o > 0 . Thi s computatio n ca n b e tackle d vi a a techniqu e simila r t o tha t o f Cartinhou r (1990) . Th e resultin g algebr a i s mess y an d i s omitted . Particularisin
g (2-13 ) gives , afte r substantia l reduction , M(t u t 2 ) = 2 exp{§(£ ? + 2Outsid
e th e norma l distributio n context , th e correlatio n i s n o longe r th e obviou s meas - ur e o f associatio n betwee n tw o variables . A recen t proposa l fo r a n alternativ e measure , especiall y relevan t i n th e contex t o f nonlinea r dependenc e amon g continuou s variables , i s th e 'correlatio n curve' , discusse d b y Bjerv e & Doksu m (1993) , whic h i s essentiall y a loca l analogu e o f th e usua l correlation , compute d fo r eac h give n valu e o f th e conditionin g variable . Thi s correlatio n curv e ca n b e readil y obtaine d fo r th e bivariat e skew-norma l distribution , b y usin g th e expression s give n i n § 3-2 . Figure
s 3 (a ) an d (b ) provid e contou r plot s o f th e bivariat e densit y (31 ) whe n 5 1 = 8 2 = 0 8 an d co i s equa l t o 0 3 an d 0-4074 , respectively . Th e valu e co = 0-407 4 correspond s t o uncorrelate d components . Additiona l plot s o f simila r typ e ar e presente d i n th e final section . 1- 0 0- 8 0 6 0- 4 0- 2 P 0 O -O 2 -0- 4 -0- 6 -0- 8 -1- 01 * =+ 1 yi - • i • i i - 1 -0- 8 -0- 6 -0- 4 -0- 2 0 0- 2 0- 4 0- 6 0- 8 1 b Fig . 1 . Relatio n between p an d 6 fo r varyin g \p.Downloaded from https://academic.oup.com/biomet/article/83/4/715/253295 by guest on 15 August 2023
722A. AZZALINI AND A. DALLA VALLE
1- 0 0- 8 0- 6 0- 4 0 2 p 0 0 -0 2 -0 4 -O 6 -O 8 -1- 0/ 1 / / - \ I/ •
- 1 -0 8 -0 6 -O 4 -0 2 0 0 2 0 4 0 6 0 8 1 Fig . 2 . Uppe r limi t (dashe d curve ) an d lowe r limi t (continuou s curve ) o f p a s a functio n o f 8 2 whe n <5 , = 06 . (a ) " =(b) . Thes e contou r plot s ar e clearl y differen t fro m ellipses , an d thei r shap e i s substantiall y influence d b y th e valu e o f co. 3-2 . The conditional distribution Th e conditiona l densit y o f Z 2 give n Z t = z x i s (3-3 ) wher e <^ c (z 2 |z 1 ; co) denote s th e conditiona l densit y associate d wit h a bivariat e norma lDownloaded from https://academic.oup.com/biomet/article/83/4/715/253295 by guest on 15 August 2023 Multivariate skew-normal distribution 723
variabl e wit h standardise d marginal s an d correlatio n . W e observ e tha t (33 ) i s a membe r o f a n extende d skew-norma l clas s o f densities , give n b y equatio n (9 ) o f Azzalin i (1985) . Th e momen t generatin g functio n o f Z 2 give n Z j i s (3-4 ) B y differentiatio n o f (3-4) , w e obtain , afte r som e algebra , th e expression s fo r th e conditiona l mea n an d variance : E(Z 2 \Z t = z t ) = a>z l var(Z 2 |Z 1 = z 1 ) = 1 - or - wher e H(x) = (x)/O( - x) denote s th e hazar d functio n o f th e standar d norma l density . 4 . SOM E EXAMPLE S WIT H REA L DAT A Th e ai m o f thi s sectio n i s t o provid e simpl e numerica l illustratio n o f th e materia l presente d s o far , focusin g o n th e bivariat e cas e t o eas e numerica l wor k an d graphica l presentation . Neithe r a ful l discussio n o f th e statistica l aspect s involve d no r a thoroug h exploratio n o f th e practica l relevanc e o f th e skew-norma l distributio n i s attempte d here . T o fit rea l data , a locatio n an d a scal e paramete r shoul d b e included . I n th e bivariat e case , th e observe d variabl e Y = (Y X , Y 2 ) T woul d b e naturall y expresse d a s Yj = X lj + k 2J Zj ( 7 = 1,2) , (4-1 ) wher e Z = (Z x , Z 2 ) T ~ SfJ^k i/0 , involvin g seve n component s t o b e estimated . Th e likelihoo d functio n i s readil y compute d fro m (3-1 ) and , fo r thes e explorator y examples , th e correspondin g estimate s hav e bee n compute d b y direc t maximisatio n o f th e log-likelihoo d itself . I t appear s howeve r preferabl e t o reparametris e (41 ) t o a s suggeste d i n Azzalin i (1985 ) fo r th e scala r case . Th e appropriatenes s o f thi s parametris - ation , a t leas t fo r th e scala r case , i s confirme d b y numerica l wor k b y Brun o Genetti , i n hi s 199
3 dissertatio n presente d a t th e Universit y o f Padua , wher e i t i s show n tha t th e lo g likelihoo d associate d wit h (4-2 ) ha s a muc h mor e regula r shap e tha n tha t associate d wit h (41) , whic h ha s lon g narro w ridges, ofte n curved . Thi s sor t o f behaviou r i s als o foun d b y Arnol d e t al . (1993) . Afte r th e likelihoo d functio n ha s bee n maximised , i t seem s howeve r preferabl e t o conver t bac k th e estimate s fro m (4-2 ) t o th e simple r for m (41) . W e shal l mak e us e o f a dat a set , collecte d b y th e Australia n Institut e o f Spor t an d reporte d b y Coo k & Weisber g (1994) , containin g severa l variable s measure d o n n = 20 2 Australia
n athletes . Specifically , w e shal l conside r th e pair s o f variable s (Wt , Ht ) an d (LBM , BMI ) wher e th e meanin g o f th e name s is : Wt , weigh t (Kg) ; Ht , heigh t (cm) ; BMI , bod y mas s inde x = Wt^Ht) 2 ; LBM , lea n bod y mass . Figur e 4 display s th e scatter-plo t o f (Wt , Ht ) wit h superimpose d contour s o f th e fitted SfJ^ distribution . Bot h th e observe d point s an d th e fitted densit y exhibi t moderat e skew - nes s fo r eac h o f th e components .Downloaded from https://academic.oup.com/biomet/article/83/4/715/253295 by guest on 15 August 2023 724A. AZZALINI AND A. DALLA VALLE
150
- 12 0 Fig . 4 . Australia n athlete s data : scatte r plo t (Wt , Ht ) an d fitted bivariat e skew - norma l distribution . A n 'obvious ' wa y o f tacklin g thes e data , an d th e othe r component s o f th e sam e dat a set , woul d b e vi a som e monotoni c transformatio n o f th e individua l components , a devic e whic h ofte n cure s nonnormalit y o f th e data ; log-transformatio n i s th e mos t commonl y employe d transformatio n fo r thi s purpose . I t ca n howeve r b e argue d tha t retainin g th e variable s o n th e origina l scal e allow s a mor e meaningfu l interpretatio n o f th e quantitie s involved . Moreover , transformatio n o f th e individua l component s ca n improv e normalit y o f th e components , bu t i t i s o f n o valu e whe n th e failur e o f normalit y refer s t o th e join t behaviou r o f th e variables . T o examin e somewha t mor e formall y whethe r th e skewnes s i s significantl y differen t fro m 0 , w e us e th e sampl e inde x o f skewnes s y t . Thi s ha s bee n show n b y Salva n (1986 ) t o b e th e locall y mos t powerfu l location-scal e invarian t statistic , fo r testin g departur e fro m normalit y withi n th e scala r ske w norma l densities . Standardisatio n o f ^ wit h it s asymptoti c standar d deviatio n (6/n) * give s 1-3 9 an d -115 , fo r W t an d Ht , respectively . Neithe
r o f the m i s significan t a t 5% , but the y ar e bot h fairl y hig h i n absolut e value . Thi s suggest s t o conside r a combine d measur e o f asymmetry , suc h a s th e inde x b li2 propose d b y Mardi a (1970 ) a s a tes t statisti c o f bivariat e normality . Fo r ou r data , th e observe d valu e o f nb 1>2 / 6 i s 56-8
, whic h i s markedl y significan t whe n compare d wit h th e xl percent - ag e points . Th e summar y conclusio n i s tha t som e departur e fro m normalit y i s present , althoug h i t i s no t eviden t i n a single-componen t analysis . Thi s i s i n conflic t wit h th e commo n belie f tha t anthropometri c measurement s ar e 'normal' , an d i s essentiall y i n agreemen t wit h th e genera l findings o f Hil l & Dixo n (1982) . Figur e 5 display s th e scatte r plo t an d th e fitted densit y fo r th e (BMI , LBM ) pair . I n thi s case , obviou s skewnes s i s presen t i n bot h components , an d th e observe d significanc e leve l o f #! , afte r standardisation , i s nearl y 0 fo r bot h components . Th e contou r leve l curve s appea r t o follo w satisfactoril y th e scatte r plot , an d the n th e fitted SfJ/~ 2 densit y seem s t o provid e a n adequat e summar y o f th e data .Downloaded from https://academic.oup.com/biomet/article/83/4/715/253295 by guest on 15 August 2023 Multivariate skew-normal distribution725
4 0 BM I Fig . 5 . Australia n athlete s data ; scatte r plo t (BMI , LBM ) an d fitted bivariat e skew - norma l distribution . ACKNOWLEDGEMEN
T W e than k Davi d Cox , To m DiCiccio , a n Associat e Edito r an d a refere e fo r a numbe r o f suggestion s whic h le d t o a bette r presentatio n o f th e material . Thi s researc h ha s bee n supporte d b y Minister o dell'Universit a e dell a Ricerc a Scientific a e Tecnologica , Italy . APPENDI
X Computation
of the density W e comput e th e densit y functio n o f Z , whos e generi c componen t i s define d b y (2-2) , a t a poin t z = (z l ,..., z k ) T e re*. Fo r subsequen t use , w e defin e w, = • an d w = (w 1; ... , w t ) T . Usin g standar d method s fo r transformation s o f rando m variables , th e den - sit y functio n o f Z a t poin t z e R * i s ui-m* x f Hrw-fr-w-^- • ex p - J>0° (1 4- )JyV~^'/
0 \ Ln >dv (A-l )Downloaded from https://academic.oup.com/biomet/article/83/4/715/253295 by guest on 15 August 2023 726 A. AZZALINI AND A. DALLA VALLE
W e recal l th e binomia l invers e theorem , whic h state s (A + UBV)- 1 = A~ 1 -A~ l UB{B fo r an y conformabl e matrices , provide d th e inverse s exist ; se e fo r instanc e Mardia , Ken t & Bibb y (1979 , p . 459)
. Usin g thi s result , w e obtai n VU-l l jTm- l (A-2 ) wher e n , define d a s i n (2-6) , i s suc h tha t Replacin
g (A-2 ) an d (A-3 ) i n (A-l) , w e obtai n (23) . REFERENCE
S AIGNER
, D . J. , LOVELL , C . A . K . & SCHMIDT , P . (1977) . Formulatio n an d estimatio n o f stochasti c frontie rproduction function model. J. Economet. 12, 21-37. ANDEL , J. , NETUKA , I . & ZVARA , K . (1984) . O n threshol d autoregressiv e processes . Kybernetika 20 , 89-106
.ARNOLD, B. C, BEAVER, R. J., GROENEVELD, R. A. & MEEKER, W. Q. (1993). The nontruncated marginal of a truncate d bivariat e norma l distribution . Psychometrica 58
, 471-8
.AZZALINI, A. (1985). A class of distributions which includes the normal ones. Scand. J. Statist. 12, 171-8.AZZALINI, A. (1986). Further results on a class of distributions which includes the normal ones. Statistica
46
, 199-208
.BJERVE, S. & DOKSUM, K. (1993). Correlation curves: measures of association as functions of covariate values.
Ann. Statist. 21
, 890-902
.CARTTNHOUR, J. (1990). One dimensional marginal density function of a truncated multivariate Normal density
function . Commun. Statist. A 19 , 197-203
. COOK , R . D . & WEISBERG , S . (1994) . An Introduction to Regression Graphics. Ne w York : Wiley .HENZE, N. (1986). A probabilistic representation of the 'skew-normal' distribution. Scand. J. Statist. 13, 271-5.HILL, M. A. & DDCON, W. J. (1982). Robustness in real life: A study of clinical laboratory data. Biometrics38, 377-96.MARDIA, K. V. (1970). Measures of multivariates skewness and kurtosis with applications. Biometrika 57,
519-30
. MARDIA
, K . V. , KENT , J . T . & BIBBY , J . M . (1979) . Multivariate Analysis. London : Academi c Press .O'HAGAN, A. & LEONARD, T. (1976). Bayes estimation subject to uncertainty about parameter constraints.
Biometrika
63
, 201-3
.SALVAN, A. (1986). Locally most powerful invariant tests of normality (in Italian). In Atti della XXXIII
Riunione
Scientifica della Societa Italiana di Statistica, 2 , pp . 173-9
. Bari : Cacucci .SCHUCANY, W. R., PARR, W. C. & BOYER, J. E. (1978). Correlation structure in Farlie-Gumbel-Morgenstern
distributions . Biometrika 65
, 650-3
.ZACKS, S. (1981). Parametric Statistical Inference. Oxford: Pergamon. [Received September 1995
. Revised March 1996
]Downloaded from https://academic.oup.com/biomet/article/83/4/715/253295 by guest on 15 August 2023