Instruction morale - Maximes
« Toute la morale est dans ce vieux proverbe : Qui mal veut mal lui arrive. » Citation de Jean-Baptiste Say - Des hommes et de la société - 1817. « Excuser le
Dynamic Multi-Context Attention Networks for Citation Forecasting of
attention mechanism for long-term citation forecasting. Ex- Citation-based bibliometrics analysis such as ... multi-head strategy to calculate ce1.
RAPPORT CE1 2017
Le sujet consistait en une citation extraite d'un texte du critique d'art. Waldemar-George qui rendait compte de sa visite à l'Exposition internationale.
Instruction civique et morale
La liberté des uns s'arrête là où commencent celle des autres. Cette expression est à la fois un précepte et un proverbe. Il apprend à restreindre ses.
Ce1–x Co x O2 Nanorods Prepared by Microwave-Assisted
6 abr. 2015 72 PUBLICATIONS 1063 CITATIONS ... 325 PUBLICATIONS 4
Pour enseigner la lecture et lécriture au CE1
Adapté aux capacités des élèves il pourra néanmoins comporter quelques difficultés qui nécessitent une expli- citation. Il sera en rapport avec la leçon d'
Cómo citar el artículo Número completo Más información del artículo
lational resources for positive youth develo- pment in conditions of adversity. The Social. Ecology of Resilence [CE1] 173-185. https://doi.
Projet pluridisciplinaire - Instruction civique et morale
Quelques productions de bandes dessinées en CE1 CE2 et CM1 Débattre sur les maximes
Effect of Ce/Zr Composition on Structure and Properties of Ce1
15 set. 2022 Citation: Pakharukova V.P.; ... Ce1?xZrxO2 Oxides and Related Ni/Ce1?xZrxO2 Catalysts for. CO2 Methanation.
Ecriture Lecture Maths Ce1 (Download Only) - accreditation.ptsem.edu
hace 5 días Thank you unconditionally much for downloading ecriture lecture maths ce1.Most likely you have knowledge that people have look numerous ...
[PDF] [PDF] 4 fiches dexercices “La phrase” - Lutin Bazar
Grammaire - CE1 La phrase Fiche n°1 ? Vérifie si ces phrases sont correctes puis entoure OUI ou NON ? Sur ton cahier recopie ces phrases en mettant
[PDF] La phrase - Exercicespdf - Le Cartable Fantastique
Exercice 1 : Entoure uniquement les phrases • La maison est trop petite • les trois petits cochons habitent avec leur maman • Très ils pauvres sont
[PDF] Je reconnais et jécris une phrase correcte - Nathan enseignants
27 mar 2020 · Nathan - Je m'exerce en grammaire - CE1 ? Colorie selon ton résultat Commec'estbizarre cettebaguetten'est plusmagique!
[PDF] La phrase - Mine de rien
Classe : CE1 EVALUATION DE GRAMMAIRE La phrase Exercice 1 : Complète Une phrase commence toujours par et se
[PDF] Mysticlolly - Fichier évaluations Grammaire CE1 CE2
tous les soirs mes parents me lisent une histoire 2 Recopie la phrase en mettant les mots dans le bon ordre Les – jouent – au – enfants – ballon
[PDF] ce1-exercices-phrasepdf - I Profs
Savoir utiliser les marques de ponctuation La phrase de la maitresse elle a la main tenu Julie avait un peu peur en arrivant ce matin
[PDF] Remets les mots dans lordre pour former une phrase - Eklablog
Place les majuscules et les points : L'ours polaire : __e suis le plus grand carnivore terrestre du monde_ __e suis tout blanc et j'ai une épaisse
La Phrase CE1 - CE2 Exercices & Évaluation à imprimer - Epopia
Leçons exercices d'évaluation sur la phrase à imprimer pour le CE1 - CE2 (PDF) La phrase CE1 - CE2 Dans notre rubrique jeux et exercices de français
Phrase - Exercices – Ce1 - Grammaire - PDF à imprimer
La phrase - Ce1 - Grammaire - Exercices • Barre les ensembles de mots qui ne forment pas une phrase • Remets les mots dans l'ordre pour écrire une phrase
Comment faire une phrase ce1 ?
Une phrase est une suite de mots qui a un sens. la phrase a un début et une fin. La phrase écrite commence toujours par une majuscule et se termine par un point.Comment mettre une phrase en ordre ?
Pour remettre les mots d'une phrase dans l'ordre, tu dois respecter les étapes suivantes :
1 Tu lis bien tous les mots pour comprendre de quoi parle la phrase.2 Tu repères le mot avec une majuscule pour trouver le début de la phrase.3 Tu repères le point pour trouver la fin de la phrase.Comment écrire une phrase correcte ?
Pour écrire une phrase, il faut :
1Se dire la phrase dans sa tête. 2Compter le nombre de mots de la phrase. 3Faire une croix pour représenter chaque mot. 4Choisir le point et le placer à la fin de la ligne de croix. 5?rire le premier mot avec une majuscule.- Une phrase est constituée d'un ou plusieurs mots, de différentes natures et fonctions, reliés entre eux. Elle commence par une majuscule et se termine par une ponctuation forte (point final, point d'exclamation, point d'interrogation, points de suspension). La phrase est organisée selon les règles de la grammaire.
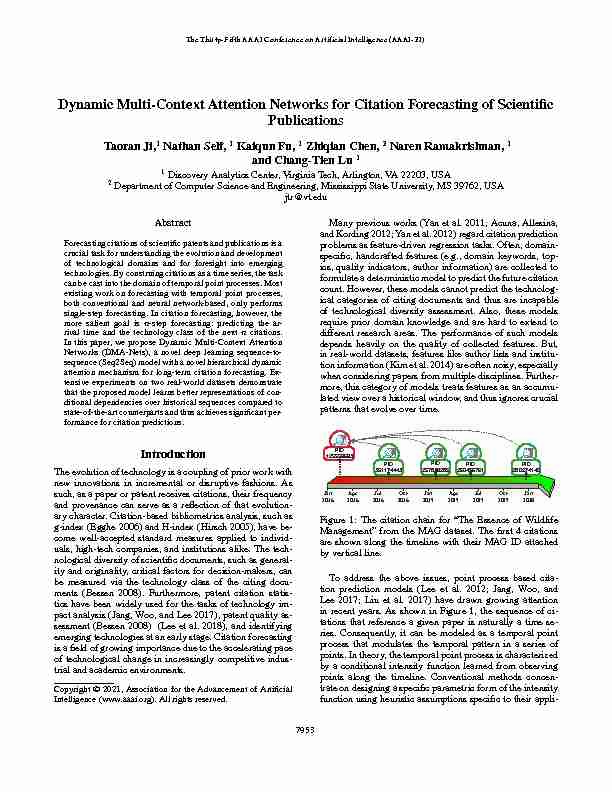
Publications
TaoranJi,
1Nathan Self,1Kaiqun Fu,1Zhiqian Chen,2NarenRamakrishnan, 1
and Chang-TienLu 1 1 DiscoveryAnalyticsCenter ,V irginiaTech, Arlington,VA22203,USA2Department ofComputer Scienceand Engineering,Mississippi StateUni versity ,MS 39762,USA
jtr@vt.eduAbstract
Forecastingcitations ofscientific patentsand publicationsis a crucial taskfor understandingthe ev olutionand development of technologicaldomains andfor foresightinto emerging technologies. Byconstruing citationsas atime series, thetask can becast intothe domainof temporalpoint processes.Most existingw orkonforecastingwith temporalpoint processes, both conventionalandneuralnetw ork-based,only performs single-step forecasting.In citation forecasting,howev er, the more salientgoa lisn-step forecasting:predicting thear - rivaltimeand thetechnology classof thene xtncitations. In thispaper ,weproposeDynamic Multi-Context Attention Networks(DMA-Nets), ano vel deeplearningsequence-to- sequence (Seq2Seq)model witha nov elhierarchical dynamic attention mechanismfor long-termcitation forecasting.Ex- tensiveexperiments ontworeal-w orlddatasets demonstrate that theproposed modellearns betterrepresentations ofcon- ditional dependencieso verhistoricalsequencescomparedto state-of-the-art counterpartsand thusachie ves significantper- formance forcitation predictions.Introduction
The evolutionoftechnologyis acoupling ofprior work with newinno vationsinincrementalordisrupti ve fashions. As such, asa paperor patentrecei ves citations,their frequency and provenancecanserve asa reflectionofthate volution- ary character.Citation-basedbibliometrics analysis,such as g-index(Egghe 2006)and H-index (Hirsch2005), havebe- come well-acceptedstandard measuresapplied toindi vid- uals, high-techcompanies, andinstitutions alike. Thetech- nological diversityofscientificdocuments, suchas general- ity andoriginality ,criticalfactors fordecision-mak ers,can be measuredviathe technologyclass ofthe citingdocu- ments (Bessen2008). Furthermore,patent citationstatis- tics havebeenwidelyused forthe tasksof technology im- pact analysis(Jang, Woo, andLee2017),patent qualityas- sessment (Bessen2008) (Leeet al.2018), andidentifying emergingtechnologies atan earlystage. Citationforecasting is afield ofgro wingimportance duetotheaccelerating pace of technologicalchange inincreasingly competitiv eindus- trial andacademic environments. Copyright© 2021,Association forthe Advancement ofArtificial Intelligence (www.aaai.org).Allrightsreserv ed.Manypre viousworks(Y anetal.2011; Acuna,Allesina, problems asfeature- drivenregressiontasks.Often,domain- specific, handcraftedfeatures (e.g.,domain ke ywords, top- ics, qualityindicators, authorinformation) arecollected to formulate adeterministic modelto predictthe future citation count. However,thesemodelscannotpredictthe technolog- ical categoriesofciting documentsand thusare incapable of technologicaldi versityassessment.Also,thesemodels require priordomain knowledge andarehardto extend to differentresearch areas.The performanceof suchmodels depends heavilyonthe qualityof collectedfeatures. But, in real-worlddatasets,features like authorlists andinstitu- tion information(Kim etal. 2014)are oftennoisy ,especially when consideringpapers frommultiple disciplines.Further - more, thi s cate gory of models treats features as an accumu- lated viewov erahistoricalwindow, andthus ignorescrucial patterns thate volveovertime.PID125559693
PID351174443
PID257839285
PID260456761PID
280274140
Jan2016Apr
2016Jul
2016Oct
2016Jan
2017Apr
2017Jul
2017Oct
2017Jan
2018Figure 1:The citationchain for"The Essenceof Wildlife Management" fromthe MAG dataset.Thefirst4 citationsare shownalongthe timelinewith theirMA GID attachedby verticalline.
Toaddress theabo ve issues,pointprocessbasedcita-
tion predictionmodels (Leeet al.2012; Jang,W oo,and Lee 2017;Liu etal. 2017)ha ve drawn growingattention in recentyears. Assho wnin Figure1,thesequence ofci- tations thatreference agi ven paperisnaturallyatimese- ries. Consequently,itcan bemodeled asa temporalpoint process thatmodulates thetemporal patternin aseries of points. Intheory ,thetemporalpoint processis characterized by aconditional intensityfunction learnedfrom observing points alongthe timeline.Con ventional methodsconcen- trate ondesigning aspecific parametricform ofthe intensity 7953cation (Mishra,Rizoiu, andXie 2016;Helmstetter andSor - nette2002).F orinstance,citationforecastingmethods(Xiao et al.2016; Liuet al.2017) usuallyfollo wthe paradigmof tensity spikeswhenev eranewcitation arriv es.Thisfeature is usedto simulatethat ahighly citedpaper ismore likely to receivemorecitations. Thesecon ventionalmethodsha ve twonotable drawbacks: (1)heuristicassumptionsmight not be ableto reectcomplicated temporaldependencies inreal datasets; and,(2) inpractice, thecomple xityof theintensity function ismathematically limited. Toaddress thechallenges conv entionalmodels havein modeling intensity,more recentapproachesuserecurrent neural networks(RNNs)( Duet al.2016;Meiand Eisner
2017) toapproximate morecomplicated conditionalinten-
sity functionswithout heuristicassumptions orprior knowl- edge ofdataset orapplication. Moste xistingRNN-based models (Wangetal. 2017;Xiao etal. 2019,2017) hav e shownimpro vedperformanceover conv entionalmethods on bothsynthetic dataand real-world datasets.RNN-based temporal pointprocess modelscan beclassied intotw o families:intensity-based modelsand end-to-endmodels. Intensity-based models(W angetal.2017; Duet al.2016) use theneural network toimplicitlymodulatethe condi- tional intensityfunction whichis thenused toobtain the conditional densityfunct ionformaximumlik elihoodesti- observationhistory but ispronetoforecasting errorpropa- gationfor thetask oflong-term prediction.The family of end-to-end models(Ji etal. 2019)combines theprocess of representing theintensity functionwith theprocess ofpre- diction. Theadv antageofend-to-endmodels isthat, with careful design,the modelcan befurther optimizedduring the predicationphase, insteadof onlyfrom theobserv ation sequence.In thispaper ,weproposean RNN-based,end-t o- end
model forcitation forecasting.This modelintroduces ahi- erarchical dynamicattention layerwhich usestw otemporal attention mechanismsto enforcethe model's abilityto rep- resent complicatedconditional dependenciesin real-world datasets andallo wthemodelto automaticallybalance the learning processfrom theobserv ationside andprediction side. Furthermore,the temporalprediction layerguaran- tees thatthe predictedcitations aremonotonically increas- ing alongthe timedimension. Specically, thecontrib utions and highlightsof thispaper are: • Formulatingano vel frameworktoprovide long-termci- tation predictionsin anend-to-end fashion byinte grating the processof learningintensity functionrepresentations and theprocess ofpredicting futurecitations. • Designingtw onovel temporalattentionmechanismsto improvethemodel' sability tomodulatecomplicatedtem- poral dependenciesand toallo wthe modeltodynamically combine theobserv ationandpredictionsides duringthe learning process. • Conductinge xtensiveexperimentsontwo real-world datasets todemonstrate thatour modelis capableof cap-turing thegeneral shapeof citationsequences andcan consistentlyoutperformothermodelsforthecitationfore-
casting task. • Curatingand releasingtw olar gedatasetsfromthe UnitedStates PatentandT rademarkOf ce(USPTO)and Mi-
crosoft AcademicGraph (MAG), whichcanbeused for citation predictiontask andgeneralized pointprocess task.ProblemF ormulation
LetC=fCigjCj
i =1be aset ofcoll ectedcitation sequencesfor scientic documents(e.g. aset ofpapers orpatents). Theith sequence isdenoted byCi=f(ti;mi)gjCij i =0wheretiandmirefer tothe publisheddate andthe technologyclass ofthe i th citation,and the0th citationis thetar getdocument itself. The citationsequencecan alsobe representedin termsof the inter-citationduration betweentwosuccessi ve citations C i=f(i;mi)gjCij i =1wherei=titi1refers tothe time differencebetween theith citationand the(i1)th citation. These tworepresentationsare equiv alent.In thispaper,we use inter-citationdurationnotation becauseit makes iteas- ier toconstrain theend-to-end modelto forecastcitations correctly alongthe timedimension suchthat ti+1ti.Givendataas describedabo ve, ourproblem isasfol-
lows:for ascient icdocument, usingtherst lcitations f (i;mi)gl i =1as observations,canwe forecastthe sequence of thene xtncitationsf(j;mj)gl+n j=l+1? Whenn= 1, the problem isa one-stepforecasting problem,which issim- pler sincelearning thetemporal pointprocess dependsonly on theobserv ationsideandthere isno errorpropag ationon the predictionside. Becausepredicting onlythe next cita- tion doesnot hav emuchpracticalvalue,we focusonly on the taskwhen n >1. Inthe casewhere n >1, thereare twochallenges forthe taskof forecastingthe next ncita- tions. First,there isa trade-off oflearning fromtheobser- vationside orfrom theprediction side.On theone hand, observationsare groundtruth but they maybetoofe wto provideenough informationto modulatethe temporalpoint process. Onthe otherhand, predictionsare lesstrustw orthy butcan provide extrainformationto themodelforlearning the temporalpoint process.Also, errorsthat occurearly in dictions. Thesechallenges motiv ateustoadoptasequence- to-sequence structurewhich takes intoaccountboththe ob- servationside andprediction sideduring thetraining.Models
By consideringthe arriv alofacitationasan instantpoint on the timeline,we canstudy theentire citationsequence asa point processwhose jointdensity functionis representedas f((1;m1);(2;m2);:::) =Y if(i;mijHi)(1) where thedensity functionat ith stepis conditionedby the information ofhistorical citationsup topoint i, denotedby H i. Inpoint processtheory ,this densityfunctionisusually learned throughthe conditionalintensity function,which is used topredict futurecitations througha generativ eprocess. 7954 Figure 2:The architecture ofDMA-Nets.L1is theinput layer.L2 isthe recurrentrepresentation layer. L3refers to the localtemporal attention(L TA) layerandL4totheglobal multi-contexttemporal attention(GMT A)layer .Together, these comprisethe dynamichierarchical attentionlayer .L5 is theprediction layer. In thispaper ,weproposea nov elframe work thatintegrates the taskof representingthe conditionalintensity function and predictingthe arriv altimeanddocumentclassof the nextncitations. Figure 2presents theo verall encoder-decoderarchitec- ture ofDMA-Nets wherethe encoderis suppliedwith the sequence ofobserv edcitationse=f(i;mi)gl i =1and the decoderaims torecurrently predictthe sequenceof the nextncitationsd=f(^j;^mj)gl+n j=l+1. Theinput layer (L1 inFig. 2)encodes temporaland category information into densev ectors.Therecurrentrepresentation layer(L2 in Fig. 2)captures thehidden dependenciesof thecurrent cita- tions overallprevious citations.The learnedrepresentations enter theattentionlayer (L3and L4in Fig.2) whichconsists of twomodules.The localtemporal attentionlayer compiles the historydependences betweeneach pairof historicalci- tations andgenerates intra-encoderstates andintra-decoder states. Next,onthe decoderside, theglobal temporalatten- tion layerfuses multipleconte xtsobtained byattendingto differentqueries onthe informationembedded bythe inner states ofboth encoderand decoder. Finally, theprediction layer (L5in Fig2) makes thetime-a warepredictionfor the nextncitations.Seq2Seq Structurefor CitationPrediction
Giventheobserv ationsequence e, ateach step,the en- coder aimsto encodeand tocompile thehidden dependen- cies acrossobserv edhistoricalcitations,thus generatinga sequence ofhidden stateshe=fhe1;:::;helg;hei2Rdh. The calculationof theith hiddenstate heiis denedin Equa- tion 2: h ei=g(aei;hei1);aei=femb(i;mi)(2) wheregis arecurrent unit,such asLSTM (Hochreiterand which capturesthe dependency structureofthecurrent inputoverthehidden stateat thepre vioussteps, andfembis theembedding layerconcatenating theembedding ofiandmito ademb-dimension densev ector.Inparticular,for theith
input(i;mi),iis rstdiscretized onyear ,month, andday, and thenis embeddedinto Rdspace, andmiis embedded into aRdmspace. Likewise,ateachstep, thedecoder takes as inputthe previous hiddenstateandthe predictionfrom the previousstep h di=g(adi;hdi1);adi=femb(^l+i;^ml+i);(3) and predictsthe waiting timeandthedocument classof next citation: ^l+i+1;^ml+i+1=p(hdi);(4) wherep()is afunction thatpredicts thene xtcitation based on thecurrent hiddenstate. Inthis work weuse anLSTM recurrent unitand employ d=dm= 32,demb=d+ d m= 64anddh= 256.HierarchicalDynamic AttentionLay er
Though recurrentneural networks havebeen successfully used inv arioustimeseriesprediction tasks (Duet al.2016), the factthatthe lasthidden stateholds theentire memory of thesequence posesa bottleneckin learning conditional dependencies acrossa longsequence oftemporal points.As a result,we proposea hierarchicaldynamic attention layer that explorespairwisetemporal dependenciesfrom bothlo- cal andglobal perspectiv esandfromtheviewpoint ofbothquotesdbs_dbs29.pdfusesText_35[PDF] citation sur l'éducation scolaire
[PDF] livre des proverbes pdf
[PDF] proverbes d'amour pdf
[PDF] proverbes francais pdf
[PDF] les proverbes africains et leurs explications pdf
[PDF] proverbes africains rigolos
[PDF] pdf citations d amour
[PDF] proverbes africains sur l amour pdf
[PDF] citation einstein intelligence pdf
[PDF] callon et latour théorie de la traduction
[PDF] citation communication interne
[PDF] callon latour coquilles saint jacques
[PDF] citation communication marketing
[PDF] citation communication relation