Ressources pour la classe de seconde - méthodes et pratiques
L’essor des sciences a donc contribué à faire évoluer l’art, tant dans ses techniques que dans ses objets traités Par ailleurs, se posent les questions de restauration et de conservation de ces œuvres L’art de la Renaissance est une thématique qui permet de montrer les liens entre science et œuvres d’arts
Mathematics and Science - NSF
sciences”, and “science” as “science, engineering, technology, medicine, business, and other applications” 2 1 Modeling Mathematical modeling, the process of describing scientific phenomena in a mathematical framework,
Recent variability of the solar spectral irradiance and its
model, the original values listed by Pagaran et al (2009) are shown As discussed by Krivova and Solanki (2013), these values should most likely be corrected by a factor of roughly 1 2 the Earth’s atmosphere, possibly also through indirect mech-anisms (e g the “top-down” mechanism, Gray et al , 2010)
NONLINEAR FORCE-FREE FIELD MODELING OF A SOLAR ACTIVE REGION
NONLINEAR FORCE-FREE FIELD MODELING OF A SOLAR ACTIVE REGION AROUND THE TIME OF A MAJOR FLARE AND CORONAL MASS EJECTION C J Schrijver,1 M L DeRosa,1 T Metcalf,2 G Barnes,2 B Lites,3 T Tarbell,1 J McTiernan,4 G Valori,5
The styrene–maleic acid copolymer: a versatile tool in
sciences MPs represent a substantial fraction of protein-encoding genes (Wallin and von Heijne 1998), they fulfill a variety of essential functions in all organisms (von Hei-jne 2007), and they have high pharmacological relevance (Overington et al 2006) Despite the evident importance of these proteins, our understanding of the principles
NEW APPROACHES TO REMOVING ALKYL-METHOXYPYRAZINES FROM GRAPE
for the taint (Pickering et al , 2005), while other MPs may contribute to a lesser extent (Botezatu et al , 2013) Importantly, the human threshold for both IPMP and IBMP in juice and wine is very low - down to 300 pg/L (Pickering et al , 2007) - meaning little is needed to compromise wine quality, and creating challenges for efforts
This page intentionally left blank - University of Rajshahi
ences, the health sciences, environmental studies, natural resource management, or other areas that make extensive use of sampling As such, we mean “survey” in the broadest sense; applications include, for instance, measured data on selected sample units, as well as responses from people to questionnaires It is written to appeal to
[PDF] science et cosmétologie enseignement d exploration
[PDF] montrer qu'une fonction est croissante terminale s
[PDF] montrer qu'une fonction est croissante seconde
[PDF] démontrer qu'une fonction est croissante sur un intervalle donné
[PDF] tp mps svt
[PDF] site de recherche de personne gratuit
[PDF] comment espionner quelqu un sur facebook
[PDF] fonction cube definition
[PDF] comment espionner quelqu un a distance
[PDF] recherche renseignement sur une personne
[PDF] tableau de signe fonction cube
[PDF] compte rendu mps seconde raisin
[PDF] mps seconde investigation policière scénario
[PDF] fonction racine cubique
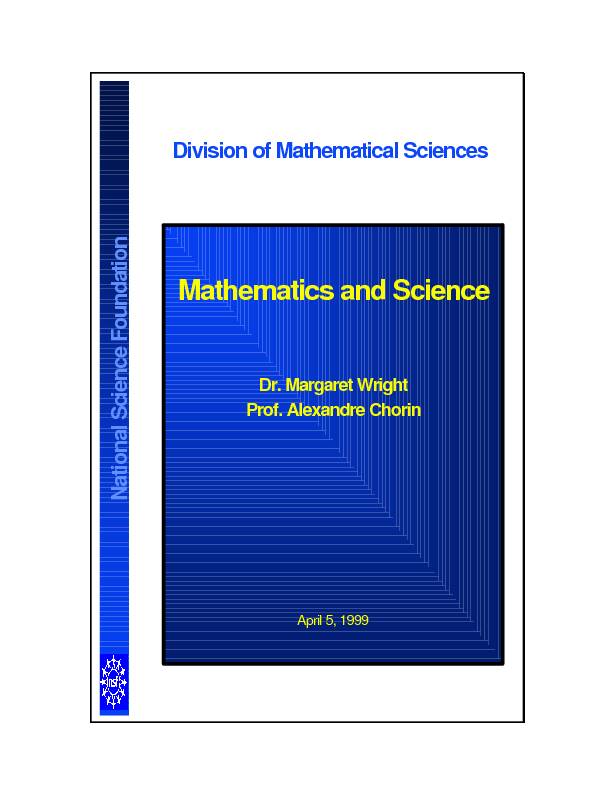
National Science Foundation
Division of Mathematical Sciences
Mathematics and Science
Dr. Margaret Wright
Prof. Alexandre Chorin
April 5, 1999
PREFACE
Today's challenges faced by science and engineering are so complex that they can only be solved through the help and participation of mathematical scientists. All three approaches to science, observation and experiment, theory, and modeling are needed to understand the complex phenomena investigated today by scientists and engineers, and each approach requires the mathematical sciences. Currently observationalists are producing enormous data sets that can only be mined and patterns discerned by the use of deep statistical and visualization tools. Indeed, there is a need to fashion new tools and, at least initially, they will need to be fashioned specifically for the data involved. Such will require the scientists, engineers, and mathematical scientists to work closely together. Scientific theory is always expressed in mathematical language. Modeling is done via the mathematical formulation using computational algorithms with the observations providing initial data for the model and serving as a check on the accuracy of the model. Modeling is used to predict behavior and in doing so validate the theory or raise new questions as to the reasonableness of the theory and often suggests the need of sharper experiments and more focused observations. Thus, observation and experiment, theory, and modeling reinforce each other and together lead to our understanding of scientific phenomena. As with data mining, the other approaches are only successful if there is close collaboration between mathematical scientists and the other disciplinarians. Dr. Margaret Wright of Bell Labs and Professor Alexandre Chorin of the University of California-Berkeley (both past and present members of the Advisory Committee for the Directorate for Mathematical and Physical Sciences) volunteered to address the need for this interplay between the mathematical sciences and other sciences and engineering in a report to the Division of Mathematical Sciences. Their report identifies six themes where there is opportunity for interaction between the mathematical sciences and other sciences and engineering, and goes one to give examples where these themes are essential for the research. These examples represent only a few of the many possibilities. Further, the report addresses the need to rethink how we train future scientists, engineers, and mathematical scientists. The report illustrates that some mathematical scientists, through collaborative efforts in research, will discover new and challenging problems. In turn, these problems will open whole new areas of research of interest and challenge to all mathematical scientists. The fundamental mathematical and statistical development of these new areas will naturally cycle back and provide new and substantial tools for attacking scientific and engineering problems. The report is exciting reading. The Division of Mathematical Sciences is greatly indebted to Dr.Wright and Professor Chorin for their effort.
Donald J. Lewis
Director (1995-1999)
Division of Mathematical Science
National Science Foundation
1 Overview
Mathematics and science
1 have a long and close relationship that is of crucial and
growing importance for both. Mathematics is an intrinsic component of science, part of its fabric, its universal language and indispensable source of intellectual tools. Reciprocally, science inspires and stimulates mathematics, posing new questions, engendering new ways of thinking, and ultimately conditioning the value system of mathematics. Fields such as physics and electrical engineering that have always been mathematical are becoming even more so. Sciences that have not been heavily mathematical in the past---for example, biology, physiology, and medicine---are moving from description and taxonomy to analysis and explanation; many of their problems involve systems that are only partially understood and are therefore inherently uncertain, demanding exploration with new mathematical tools. Outside the traditional spheres of science and engineering, mathematics is being called upon to analyze and solve a widening array of problems in communication, finance, manufacturing, and business. Progress in science, in all its branches, requires close involvement and strengthening of the mathematical enterprise; new science and new mathematics go hand in hand. The present document cannot be an exhaustive survey of the interactions between mathematics and science. Its purpose is to present examples of scientific advances made possible by a close interaction between science and mathematics, and draw conclusions whose validity should transcend the examples. We have labeled the examples by words that describe their scientific content; we could have chosen to use mathematical categories and reached the very same conclusions. A section labeled "partial differential equations" would have described their roles in combustion, cosmology, finance, hybrid system theory, Internet analysis, materials science, mixing, physiology, iterative control, and moving boundaries; a section on statistics would have described its contributions to the analysis of the massive data sets associated with cosmology, finance, functional MRI, and the Internet; and a section on computation would have conveyed its key role in all areas of science. This alternative would have highlighted the mathematical virtues of generality and abstraction; the approach we have taken emphasizes the ubiquity and centrality of mathematics from the point of view of science.2 Themes
As Section 3 illustrates, certain themes consistently emerge in the closest relationships between mathematics and science:· modeling
· complexity and size
· uncertainty
· multiple scales
· computation
· large data sets.
1 For compactness, throughout this document "mathematics" should be interpreted as "the mathematicalsciences", and "science" as "science, engineering, technology, medicine, business, and other applications".
2.1 Modeling
Mathematical modeling, the process of describing scientific phenomena in a mathematical framework,brings the powerful machinery of mathematics---its ability to generalize, to extract what is common in
diverse problems, and to build effective algorithms---to bear on characterization, analysis, and prediction in
scientific problems. Mathematical models lead to "virtual experiments" whose real-world analogues would
be expensive, dangerous, or even impossible; they obviate the need to actually crash an airplane, spread a
deadly virus, or witness the origin of the universe. Mathematical models help to clarify relationships
among a system's components as well as their relative significance. Through modeling, speculations about
a system are given a form that allows them to be examined qualitatively and quantitatively from many angles; in particular, modeling allows the detection of discrepancies between theory and reality.2.2 Complexity and Size
Because reality is almost never simple, there is constant demand for more complex models. However, ever more complex models lead eventually---sometimes immediately- --to problems that are fundamentally different, not just larger and more complicated. It is impossible to characterize disordered systems with the very same tools that are perfectly adequate for well-behaved systems. Size can be regarded as a manifestation of complexity because substantially larger models seldom behave like expanded versions of smaller models; large chaotic systems cannot be described in the same terms as small- dimensional chaotic systems.2.3 Uncertainty
Although uncertainty is unavoidable, ignoring it can be justified when one is studying isolated, small-scale, well-understood physical processes. This is not so for large-scale systems with many components, such as the atmosphere and the oceans, chemical processes where there is no good way to determine reaction paths exactly, and of course in biological and medical applications, or in systems that rely on human participation. Uncertainty cannot be treated properly using ad hoc rules of thumb, but requires serious mathematical study. Issues that require further analysis include: the correct classification of the various ways in which uncertainty affects mathematical models; the sensitivities to uncertainty of both the models and the methods of analysis; the influence of uncertainty on computing methods; and the interactions between uncertainty in the models themselves and the added uncertainty arising from the limitations of computers. Uncertainty of outcome is not necessarily directly related to uncertainty in the system or in the model. Very noisy systems can give rise to reliable outcomes, and in such cases it is desirable to know how these outcomes arise and how to predict them. Another extreme can occur with strongly chaotic systems: even if a specific solution of a model can be found, the probability that it will actually be observed may be nil; thus it may be necessary to predict the average outcome of computations or experiments, or the most likely outcome, drawing on as yet untapped resources of statistics.2.4 Multiple Scales
The need to model or compute on multiple scales arises when occurrences on vastly disparate scales (in
space, time, or both) contribute simultaneously to an observable outcome. In turbulent combustion, for
example, the shape of the vessel is important and so are the very small fluctuations in temperature that
control the chemical reactions. Multiple scales are inherent in complex systems, a topic of greatimportance across science, whenever entities at microscales and macrolevels must be considered together.
When it is known in advance that phenomena on different scales are independent, one may rely on a separate model on each scale; but when different scales interact, or when the boundaries between scales become blurred, models are needed that allow interactions between scales without an undue sacrifice of structure or loss of information at any scale. A related complication is that the finiteness of computers limits the range of scales that can be represented in a given calculation; only mathematical analysis can overcome this built-in restriction.2.5 Computation
Experiment and theory, the two classical elements of the scientific method, have been joined bycomputation as a third crucial component. Computations that were intractable even a few years ago are
performed routinely today, and many people pin their hopes for mastering problem size and complexity on
the continuing advent of faster, larger computers. This is a vain hope if the appropriate mathematics is
lacking. For more than 40 years, gains in problem-solving power from better mathematical algorithmshave been comparable to the growth of raw computing speed, and this pattern is likely to continue. In
many situations, especially for multiscale and chaotic problems, fast hardware alone will never besufficient; methods and theories must be developed that can extract the best possible numerical solutions
from whatever computers are available. It is important to remember that no amount of computing power or storage can overcome uncertainties in equations and data; computed solutions cannot be understood properly unless the right mathematical tools are used. A striking visualization produced over many days of computation is just a pretty picture if there are flaws in the underlying mathematical model or numerical methods, or if there are no good ways to represent, manipulate, and analyze the associated data. It is also worthy of note that computation has come to permeate even the traditional core mathematical areas, which allot expanding roles for computation, both numerical and symbolic.