Logarithmic Sobolev Inequalities
logarithmic Sobolev inequalities what they are some history analytic
Logsobwpause
Logarithmic Sobolev Inequalities
LOGARITHMIC SOBOLEV INEQUALITIES. By LEONARD GRoss.*. 1. Introduction. Classical Sobolev inequalities state typically
Lectures on Logarithmic Sobolev Inequalities
Jan 1 2012 Section 4.1 Properties of logarithmic Sobolev inequality ... ing to learn how to prove log-Sobolev inequality in infinite-dimensional ...
SPS
1 CONCENTRATION OF MEASURE AND LOGARITHMIC
2.2 Examples of logarithmic Sobolev inequalities p. 26. 2.3 The Herbst argument p. 29. 2.4 Entropy-energy inequalities and non-Gaussian tails.
Berlin
Mass transport and variants of the logarithmic Sobolev inequality
Sep 25 2007 Abstract. We develop the optimal transportation approach to modified log-Sobolev inequalities and to isoperimetric inequalities.
Modified Log-Sobolev Inequalities Mixing and Hypercontractivity
These inequalities turn out to be weaker than the stan- dard log-Sobolev inequality but stronger than the Poincare'. (spectral gap) inequality.
STOC BT
Concentration of measure and logarithmic Sobolev inequalities
4.3 Poincare inequalities and modified logarithmic Sobolev inequalities 5.1 Logarithmic Sobolev inequality for Bernoulli and Poisson measures.
SPS
Logarithmic Sobolev inequalities for unbounded spin systems revisited
or logarithmic Sobolev inequality and to control the dependence of the Logarithmic Sobolev inequalities for compact spin systems have been studied.
SPS
Logarithmic Sobolev Inequalities Essentials: Probabilistic Side
Logarithmic Sobolev Inequalities Essentials: Probabilistic Side. High dimensional probability. Rough lecture notes. Djalil Chafaï & Joseph Lehec.
chafai lehec m lsie lecture notes
Analytic and Geometric Logarithmic Sobolev Inequalities
One basic form of the logarithmic Sobolev inequality is the one for the standard. Gaussian probability measure dµ(x) = (2π)−n/2 e−
jedp.
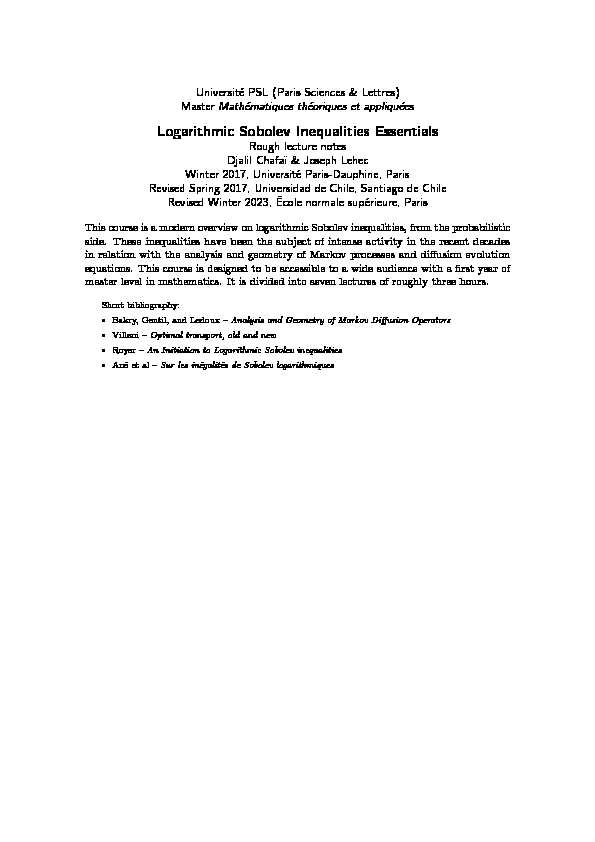
Université PSL (Paris Sciences & Lettres)
MasterMathématiques théoriques et appliquéesLogarithmic Sobolev Inequalities Essentials
Rough lecture notes
Djalil Chafaï & Joseph Lehec
Winter 2017, Université Paris-Dauphine, Paris
Revised Spring 2017, Universidad de Chile, Santiago de ChileRevised Winter 2023, École normale supérieure, ParisThis course is a modern overview on logarithmic Sobolev inequalities, from the probabilistic
side. These inequalities have been the subject of intense activity in the recent decades in relation with the analysis and geometry of Markov processes and diffusion evolution equations. This course is designed to be accessible to a wide audience with a first year of master level in mathematics. It is divided into seven lectures of roughly three hours.Short bibliography:
•Bakry, Gentil, and Ledoux -Analysis and Geometry of Markov Diffusion Operators •Villani -Optimal transport, old and new •Royer -An Initiation to Logarithmic Sobolev inequalities •Ané et al -Sur les inégalités de Sobolev logarithmiques 2Contents
1 Introduction5
1.1 Generalities on Markov processes . . . . . . . . . . . . . . . . . . . . . . . . . . . . 6
1.2 Ornstein-Uhlenbeck semigroup . . . . . . . . . . . . . . . . . . . . . . . . . . . . . 8
1.3 Poincaré and logarithmic Sobolev inequalities . . . . . . . . . . . . . . . . . . . . . 11
1.4 Convergence to equilibrium . . . . . . . . . . . . . . . . . . . . . . . . . . . . . . . 14
1.5 Amnesia and long time behavior . . . . . . . . . . . . . . . . . . . . . . . . . . . . 15
1.6 Tensorization and Central Limit Theorem . . . . . . . . . . . . . . . . . . . . . . . 17
2 Hypercontractivity, spectral gap, information theory 21
2.1 Hypercontractivity . . . . . . . . . . . . . . . . . . . . . . . . . . . . . . . . . . . . 21
2.2 Spectral gap and Hermite polynomials . . . . . . . . . . . . . . . . . . . . . . . . . 24
2.3 Information theory . . . . . . . . . . . . . . . . . . . . . . . . . . . . . . . . . . . . 28
3 Sub-Gaussian concentration and transportation 35
3.1 Concentration of measure . . . . . . . . . . . . . . . . . . . . . . . . . . . . . . . . 35
3.2 Transportation inequalities . . . . . . . . . . . . . . . . . . . . . . . . . . . . . . . 37
4 Isoperimetric inequalities 45
4.1 Proof of the second Bobkov inequality . . . . . . . . . . . . . . . . . . . . . . . . . 51
5 Bakry-Émery criterion 53
5.1 Gamma calculus . . . . . . . . . . . . . . . . . . . . . . . . . . . . . . . . . . . . . 53
5.2 Poincaré inequality . . . . . . . . . . . . . . . . . . . . . . . . . . . . . . . . . . . . 55
5.3 Langevin semigroup . . . . . . . . . . . . . . . . . . . . . . . . . . . . . . . . . . . 57
5.4 Diffusions . . . . . . . . . . . . . . . . . . . . . . . . . . . . . . . . . . . . . . . . . 58
5.5 Log-Sobolev inequality for a diffusion . . . . . . . . . . . . . . . . . . . . . . . . . 60
5.6 Kannan-Lovász-Simonovits conjecture . . . . . . . . . . . . . . . . . . . . . . . . 62
6 Brenier and Caffarelli theorems 65
6.1 Brenier theorem . . . . . . . . . . . . . . . . . . . . . . . . . . . . . . . . . . . . . 65
6.2 Monge-Ampère equation . . . . . . . . . . . . . . . . . . . . . . . . . . . . . . . . 66
6.3 Caffarelli contraction theorem . . . . . . . . . . . . . . . . . . . . . . . . . . . . . . 67
6.4 From Monge-Ampère to Gaussian log-Sobolev . . . . . . . . . . . . . . . . . . . . 69
7 Discrete space71
7.1 Bernoulli distributions . . . . . . . . . . . . . . . . . . . . . . . . . . . . . . . . . . 71
7.2 Poisson distributions . . . . . . . . . . . . . . . . . . . . . . . . . . . . . . . . . . . 73
7.2.1 Poisson process andAmodified inequality . . . . . . . . . . . . . . . . . . . 76
7.2.2 M/M/∞queue andBmodified inequality . . . . . . . . . . . . . . . . . . . 76
7.2.3 Concentration andCmodified inequality . . . . . . . . . . . . . . . . . . . 78
34CONTENTS
Chapter 1
IntroductionThe first chapters focus on a Gaussian model formed with the product spaceRnequipped with the Gaussian probability measureγn=γ?n1. It allows explicit computations. It appears asympotically in other models due to the central limit phenomenon1, in particular from spheres and from cubes, both equipped with the uniform measure. In a wayγnplays the role of a uniform measure onRn. The standard Gaussian measureγnonRnhas expectation0, covariance the identity matrixIn, and density with respect to the Lebesgue measure given by x?→(2π)-n/2e-|x|2/2 where|x|=??1/2denotes the Euclidean norm.
LetUnbe the uniform distribution on the unit sphere{s?Rn:|s|= 1}. Theorem 1.1(Polar factorization of spheres).IfX= (X1,...,Xn)≂γnthen |X|=?X21+···+X2nandX|X|
are independent, with|X|2≂χ2(n)andX/|X| ≂ Un. Conversely ifRandUare indepen- dent withR2≂χ2(n)andU≂ Un, thenRU≂γn. Proof.Follows frome-|x|2/2dx= e-r2/2rn-1drduwherex=ru. The following result, known as the Borel or Poincaré observation, states that one can see the standard Gaussian as a the projection of the uniform distribution on high dimensional spheres with radius equal to the square root of the dimension. Theorem 1.2(Central Limit Theorem for spheres).IfUn≂ Unfor anyn≥1, then for any fixedk≥1,proj(⎷nU n,Rk)→γkin law asn→ ∞.Proof.
Let(Xn)n≥1be independent and identically distributed random variables with law γ1. By the preceding theorem and the strong law of large numbers, proj( ⎷nU n,Rk)d=⎷n (X1,...,Xk)? X21+···+X2na.s.
-→n→∞(X1,...,Xk)≂γk.1IfX1,X2,...are i.i.d. real random variables with zero mean and unit variance thenX1+···+Xn⎷nconverges
in law asn→ ∞to the standard Gaussian distribution. 56CHAPTER 1. INTRODUCTION
Definition 1.3(Variance and entropy).Iffis a square integrable function with respect toγn, then itsvarianceis Varγn(f) =?
R nf2dγn-? R nfdγn? 2 = Var(f(X))whereX≂γn. Iffis a non negative function, integrable with respect toγn, then itsentropyis Entγn(f) =?
R nflogfdγn-? R nfdγn? log? R nfdγn? .The functionflogfmay not be integrable, but since the functionxlogxis bounded from below, the integral offlogfalways makes sense inR? {+∞}. Remark(φentropies).One can define a more general object: Given a convex functionφ on an intervalI?Rtheφ-entropy off:R→Iis E n(f) =? R nφ(f)dγn-φ? R nfdγn? We recover the variance forφ(x) =x2andI=Rand the entropy forφ(x) =xlog(x)andUniversité PSL (Paris Sciences & Lettres)
MasterMathématiques théoriques et appliquéesLogarithmic Sobolev Inequalities Essentials
Rough lecture notes
Djalil Chafaï & Joseph Lehec
Winter 2017, Université Paris-Dauphine, Paris
Revised Spring 2017, Universidad de Chile, Santiago de ChileRevised Winter 2023, École normale supérieure, ParisThis course is a modern overview on logarithmic Sobolev inequalities, from the probabilistic
side. These inequalities have been the subject of intense activity in the recent decades in relation with the analysis and geometry of Markov processes and diffusion evolution equations. This course is designed to be accessible to a wide audience with a first year of master level in mathematics. It is divided into seven lectures of roughly three hours.Short bibliography:
•Bakry, Gentil, and Ledoux -Analysis and Geometry of Markov Diffusion Operators •Villani -Optimal transport, old and new •Royer -An Initiation to Logarithmic Sobolev inequalities •Ané et al -Sur les inégalités de Sobolev logarithmiques 2Contents
1 Introduction5
1.1 Generalities on Markov processes . . . . . . . . . . . . . . . . . . . . . . . . . . . . 6
1.2 Ornstein-Uhlenbeck semigroup . . . . . . . . . . . . . . . . . . . . . . . . . . . . . 8
1.3 Poincaré and logarithmic Sobolev inequalities . . . . . . . . . . . . . . . . . . . . . 11
1.4 Convergence to equilibrium . . . . . . . . . . . . . . . . . . . . . . . . . . . . . . . 14
1.5 Amnesia and long time behavior . . . . . . . . . . . . . . . . . . . . . . . . . . . . 15
1.6 Tensorization and Central Limit Theorem . . . . . . . . . . . . . . . . . . . . . . . 17
2 Hypercontractivity, spectral gap, information theory 21
2.1 Hypercontractivity . . . . . . . . . . . . . . . . . . . . . . . . . . . . . . . . . . . . 21
2.2 Spectral gap and Hermite polynomials . . . . . . . . . . . . . . . . . . . . . . . . . 24
2.3 Information theory . . . . . . . . . . . . . . . . . . . . . . . . . . . . . . . . . . . . 28
3 Sub-Gaussian concentration and transportation 35
3.1 Concentration of measure . . . . . . . . . . . . . . . . . . . . . . . . . . . . . . . . 35
3.2 Transportation inequalities . . . . . . . . . . . . . . . . . . . . . . . . . . . . . . . 37
4 Isoperimetric inequalities 45
4.1 Proof of the second Bobkov inequality . . . . . . . . . . . . . . . . . . . . . . . . . 51
5 Bakry-Émery criterion 53
5.1 Gamma calculus . . . . . . . . . . . . . . . . . . . . . . . . . . . . . . . . . . . . . 53
5.2 Poincaré inequality . . . . . . . . . . . . . . . . . . . . . . . . . . . . . . . . . . . . 55
5.3 Langevin semigroup . . . . . . . . . . . . . . . . . . . . . . . . . . . . . . . . . . . 57
5.4 Diffusions . . . . . . . . . . . . . . . . . . . . . . . . . . . . . . . . . . . . . . . . . 58
5.5 Log-Sobolev inequality for a diffusion . . . . . . . . . . . . . . . . . . . . . . . . . 60
5.6 Kannan-Lovász-Simonovits conjecture . . . . . . . . . . . . . . . . . . . . . . . . 62
6 Brenier and Caffarelli theorems 65
6.1 Brenier theorem . . . . . . . . . . . . . . . . . . . . . . . . . . . . . . . . . . . . . 65
6.2 Monge-Ampère equation . . . . . . . . . . . . . . . . . . . . . . . . . . . . . . . . 66
6.3 Caffarelli contraction theorem . . . . . . . . . . . . . . . . . . . . . . . . . . . . . . 67
6.4 From Monge-Ampère to Gaussian log-Sobolev . . . . . . . . . . . . . . . . . . . . 69
7 Discrete space71
7.1 Bernoulli distributions . . . . . . . . . . . . . . . . . . . . . . . . . . . . . . . . . . 71
7.2 Poisson distributions . . . . . . . . . . . . . . . . . . . . . . . . . . . . . . . . . . . 73
7.2.1 Poisson process andAmodified inequality . . . . . . . . . . . . . . . . . . . 76
7.2.2 M/M/∞queue andBmodified inequality . . . . . . . . . . . . . . . . . . . 76
7.2.3 Concentration andCmodified inequality . . . . . . . . . . . . . . . . . . . 78
34CONTENTS
Chapter 1
IntroductionThe first chapters focus on a Gaussian model formed with the product spaceRnequipped with the Gaussian probability measureγn=γ?n1. It allows explicit computations. It appears asympotically in other models due to the central limit phenomenon1, in particular from spheres and from cubes, both equipped with the uniform measure. In a wayγnplays the role of a uniform measure onRn. The standard Gaussian measureγnonRnhas expectation0, covariance the identity matrixIn, and density with respect to the Lebesgue measure given by x?→(2π)-n/2e-|x|2/2 where|x|=??1/2denotes the Euclidean norm.
LetUnbe the uniform distribution on the unit sphere{s?Rn:|s|= 1}. Theorem 1.1(Polar factorization of spheres).IfX= (X1,...,Xn)≂γnthen |X|=?X21+···+X2nandX|X|
are independent, with|X|2≂χ2(n)andX/|X| ≂ Un. Conversely ifRandUare indepen- dent withR2≂χ2(n)andU≂ Un, thenRU≂γn. Proof.Follows frome-|x|2/2dx= e-r2/2rn-1drduwherex=ru. The following result, known as the Borel or Poincaré observation, states that one can see the standard Gaussian as a the projection of the uniform distribution on high dimensional spheres with radius equal to the square root of the dimension. Theorem 1.2(Central Limit Theorem for spheres).IfUn≂ Unfor anyn≥1, then for any fixedk≥1,proj(⎷nU n,Rk)→γkin law asn→ ∞.Proof.
Let(Xn)n≥1be independent and identically distributed random variables with law γ1. By the preceding theorem and the strong law of large numbers, proj( ⎷nU n,Rk)d=⎷n (X1,...,Xk)? X21+···+X2na.s.
-→n→∞(X1,...,Xk)≂γk.1IfX1,X2,...are i.i.d. real random variables with zero mean and unit variance thenX1+···+Xn⎷nconverges
in law asn→ ∞to the standard Gaussian distribution. 56CHAPTER 1. INTRODUCTION
Definition 1.3(Variance and entropy).Iffis a square integrable function with respect toγn, then itsvarianceis Varγn(f) =?
R nf2dγn-? R nfdγn? 2 = Var(f(X))whereX≂γn. Iffis a non negative function, integrable with respect toγn, then itsentropyis Entγn(f) =?
R nflogfdγn-? R nfdγn? log? R nfdγn? .The functionflogfmay not be integrable, but since the functionxlogxis bounded from below, the integral offlogfalways makes sense inR? {+∞}. Remark(φentropies).One can define a more general object: Given a convex functionφ on an intervalI?Rtheφ-entropy off:R→Iis E n(f) =? R nφ(f)dγn-φ? R nfdγn? We recover the variance forφ(x) =x2andI=Rand the entropy forφ(x) =xlog(x)and- log-sobolev inequality for the continuum sine-gordon model
- log sobolev inequality proof
- logarithmic sobolev inequality
- log-sobolev inequalities
- logarithmic sobolev inequalities gross
- logarithmic sobolev inequalities on noncompact riemannian manifolds
- logarithmic sobolev inequalities conditions and counterexamples
- gaussian logarithmic sobolev inequality