Acces PDF Transforming Variables For Normality And Sas Support
6 days ago Transforming Data - Data Analysis with R log Transform R SPSS Tutorial: Transforming asymmetrical/skewed data. Transforming a right skewed ...
Data pre-processing for k- means clustering
Data transformations to manage skewness. Logarithmic transformation (positive values only) import numpy as np frequency_log= np.log(datamart['Frequency']).
chapter
Kriging on highly skewed data for DTPA-extractable soil Zn with
Keywords: Skewed distribution; Transformation; Zinc availability; Ordinary kriging; Log-normal; Rank order; Normal score; Cokriging; Auxiliary variables.
LambertW: Probabilistic Models to Analyze and Gaussianize Heavy
The transformed RV Y has a Lambert W x F distribution. This package contains functions to model and analyze skewed heavy-tailed data the Lambert Way:.
LambertW
Week 7: Cost data and Generalized Linear Models
Log transformation. The most common transformation –the knee-jerk transformation– with skewed data is to use ln(y) (called log-level model since we leave
Transformations and outliers
sensitive to outliers and strongly affected by skewed data We have already seen one example of a log transform
Too many zeros and/or highly skewed? A tutorial on modelling
Jun 22 2020 strategies for this data involve explicit (or implied) transformations. (smoker v. non-smoker
Explorations in statistics: the log transformation
conform to a skewed distribution then a log transformation can make Log.R and the data file Table_1_Data.csv4 to your Advances.
Preferring Box-Cox transformation instead of log transformation to
Apr 14 2022 Conclusion: When the data is skewed
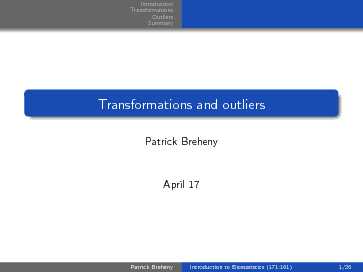
Introduction
Transformations
Outliers
SummaryTransformations and outliers
Patrick Breheny
April 17
Patrick BrehenyIntroduction to Biostatistics (171:161) 1/26Introduction
Transformations
Outliers
SummaryProblems witht-testsIn the last lecture, we covered the standard way of analyzing whether or not a continuous outcome is dierent between twogroups: thet-testHowever, the focus of thet-test is entirely upon the meanAs you may recall from our lecture on descriptive statistics
towards the beginning of the course, the mean is verysensitive to outliers, and strongly aected by skewed dataIn cases where the mean is an unreliable measure of central
tendency, thet-test will be an unreliable test of dierences in central tendencies Patrick BrehenyIntroduction to Biostatistics (171:161) 2/26Introduction
Transformations
Outliers
SummaryTransforming the data
When it comes to skewed distributions, the most common response is to transform the dataGenerally, the most common type of skewness is right-skewnessConsequently, the most common type of transformation is the log transformWe have already seen one example of a log transform, when we found a condence interval for the log odds ratio instead of the odds ratio Patrick BrehenyIntroduction to Biostatistics (171:161) 3/26Introduction
Transformations
Outliers
SummaryExample: Triglyceride levels
As an example of the log transform, consider the levels of triglycerides in the blood of individuals, as measured in theNHANES study:TRG
Frequency
100200300400
0 200400
600
800
1000
TRG
Frequency
0 100200
300
400
500
600
700
163264128256512Patrick BrehenyIntroduction to Biostatistics (171:161) 4/26
Introduction
Transformations
Outliers
SummaryLow-carb diet study
Putting this observation into practice, let's consider a 2003 study published in theNew England Journal of Medicineof whether low-carbohydrate diets are eective at reducing serum triglyceride levelsThe investigators studied overweight individuals for six months, randomly assigning one group to a low-fat diet and another group to a low-carb dietOne of the outcomes of interest was the reduction in triglyceride levels over the course of the study Patrick BrehenyIntroduction to Biostatistics (171:161) 5/26Introduction
Transformations
Outliers
SummaryAnalysis of untransformed data
The group on the low-fat diet reduced their triglyceride levels by an average of 7 mg/dl, compared with 38 for the low-carb groupThe pooled standard deviation was 66 mg/dl, and the samplesizes were 43 and 36, respectivelyThus,SE= 66p1=43 + 1=36 = 15The dierence between the means is therefore31=15 = 2:08
standard errors away from the expected value under the nullThis produces the moderately signicantp-value (p=:04)Patrick BrehenyIntroduction to Biostatistics (171:161) 6/26
Introduction
Transformations
Outliers
SummaryAnalysis of transformed data
On the other hand, let's analyze the log-transformed data Looking at log-triglyceride levels, the group on the low-fat diet saw an average reduction of 1.8, compared with 3.5 for the low-carb groupThe pooled standard deviation of the log-triglyceride levels was 2.2Thus,SE= 2:2p1=43 + 1=36 = 0:5The dierence between the means is therefore1:7=0:5 = 3:4standard errors away from the expected value under the nullThis produces a much more powerful analysis:p=:001Patrick BrehenyIntroduction to Biostatistics (171:161) 7/26
Introduction
Transformations
Outliers
SummaryCondence intervals
It's also worth discussing the implications of transformations on condence intervalsThe (Student's) condence interval for the dierence in log-triglyceride levels is3:51:81:99(0:5) = (0:71;2:69); this is fairly straightforwardBut what does this mean in terms of the original units: triglyceride levels?Recall that dierences on the log scale are ratios on the original scale; thus, when we invert the transformation (by exponentiating, also known as taking the \antilog"), we will obtain a condence interval for the ratio between the two means Patrick BrehenyIntroduction to Biostatistics (171:161) 8/26Introduction
Transformations
Outliers
SummaryCondence intervals (cont'd)
Thus, in the low-carb diet study, we see a dierence of 1.7 on the log scale; this corresponds to a ratio ofe1:7= 5:5on the original scale { in other words, subjects on the low-carb diet reduced their triglycerides 5.5 times more than subjects on the low-fat dietSimilarly, to calculate a condence interval, we exponentiate the two endpoints (note the similarity to constructing CIs for the odds ratio): (e0:71;e2:69) = (2;15)NOTE: The mean of the log-transformed values is not the same as the log of the mean. The (exponentiated) mean of the log-transformed values is known as thegeometric mean. What we have actually constructed a condence interval for is the ratio of the geometric means. Patrick BrehenyIntroduction to Biostatistics (171:161) 9/26Introduction
Transformations
Outliers
SummaryThe big picture
If the data looks relatively normal after the transformation, wecan simply perform at-test on the transformed observationsThet-test assumes a normal distribution, so this
transformation will generally result in a more powerful, lessIntroduction
Transformations
Outliers
SummaryTransformations and outliers
Patrick Breheny
April 17
Patrick BrehenyIntroduction to Biostatistics (171:161) 1/26Introduction
Transformations
Outliers
SummaryProblems witht-testsIn the last lecture, we covered the standard way of analyzing whether or not a continuous outcome is dierent between twogroups: thet-testHowever, the focus of thet-test is entirely upon the meanAs you may recall from our lecture on descriptive statistics
towards the beginning of the course, the mean is verysensitive to outliers, and strongly aected by skewed dataIn cases where the mean is an unreliable measure of central
tendency, thet-test will be an unreliable test of dierences in central tendencies Patrick BrehenyIntroduction to Biostatistics (171:161) 2/26Introduction
Transformations
Outliers
SummaryTransforming the data
When it comes to skewed distributions, the most common response is to transform the dataGenerally, the most common type of skewness is right-skewnessConsequently, the most common type of transformation is the log transformWe have already seen one example of a log transform, when we found a condence interval for the log odds ratio instead of the odds ratio Patrick BrehenyIntroduction to Biostatistics (171:161) 3/26Introduction
Transformations
Outliers
SummaryExample: Triglyceride levels
As an example of the log transform, consider the levels of triglycerides in the blood of individuals, as measured in theNHANES study:TRG
Frequency
100200300400
0 200400
600
800
1000
TRG
Frequency
0 100200
300
400
500
600
700
163264128256512Patrick BrehenyIntroduction to Biostatistics (171:161) 4/26
Introduction
Transformations
Outliers
SummaryLow-carb diet study
Putting this observation into practice, let's consider a 2003 study published in theNew England Journal of Medicineof whether low-carbohydrate diets are eective at reducing serum triglyceride levelsThe investigators studied overweight individuals for six months, randomly assigning one group to a low-fat diet and another group to a low-carb dietOne of the outcomes of interest was the reduction in triglyceride levels over the course of the study Patrick BrehenyIntroduction to Biostatistics (171:161) 5/26Introduction
Transformations
Outliers
SummaryAnalysis of untransformed data
The group on the low-fat diet reduced their triglyceride levels by an average of 7 mg/dl, compared with 38 for the low-carb groupThe pooled standard deviation was 66 mg/dl, and the samplesizes were 43 and 36, respectivelyThus,SE= 66p1=43 + 1=36 = 15The dierence between the means is therefore31=15 = 2:08
standard errors away from the expected value under the nullThis produces the moderately signicantp-value (p=:04)Patrick BrehenyIntroduction to Biostatistics (171:161) 6/26
Introduction
Transformations
Outliers
SummaryAnalysis of transformed data
On the other hand, let's analyze the log-transformed data Looking at log-triglyceride levels, the group on the low-fat diet saw an average reduction of 1.8, compared with 3.5 for the low-carb groupThe pooled standard deviation of the log-triglyceride levels was 2.2Thus,SE= 2:2p1=43 + 1=36 = 0:5The dierence between the means is therefore1:7=0:5 = 3:4standard errors away from the expected value under the nullThis produces a much more powerful analysis:p=:001Patrick BrehenyIntroduction to Biostatistics (171:161) 7/26
Introduction
Transformations
Outliers
SummaryCondence intervals
It's also worth discussing the implications of transformations on condence intervalsThe (Student's) condence interval for the dierence in log-triglyceride levels is3:51:81:99(0:5) = (0:71;2:69); this is fairly straightforwardBut what does this mean in terms of the original units: triglyceride levels?Recall that dierences on the log scale are ratios on the original scale; thus, when we invert the transformation (by exponentiating, also known as taking the \antilog"), we will obtain a condence interval for the ratio between the two means Patrick BrehenyIntroduction to Biostatistics (171:161) 8/26Introduction
Transformations
Outliers
SummaryCondence intervals (cont'd)
Thus, in the low-carb diet study, we see a dierence of 1.7 on the log scale; this corresponds to a ratio ofe1:7= 5:5on the original scale { in other words, subjects on the low-carb diet reduced their triglycerides 5.5 times more than subjects on the low-fat dietSimilarly, to calculate a condence interval, we exponentiate the two endpoints (note the similarity to constructing CIs for the odds ratio): (e0:71;e2:69) = (2;15)NOTE: The mean of the log-transformed values is not the same as the log of the mean. The (exponentiated) mean of the log-transformed values is known as thegeometric mean. What we have actually constructed a condence interval for is the ratio of the geometric means. Patrick BrehenyIntroduction to Biostatistics (171:161) 9/26Introduction
Transformations
Outliers
SummaryThe big picture
If the data looks relatively normal after the transformation, wecan simply perform at-test on the transformed observationsThet-test assumes a normal distribution, so this
transformation will generally result in a more powerful, less- log transformation for right skewed data