Acces PDF Transforming Variables For Normality And Sas Support
il y a 6 jours normality and data transformation in SPSS ... How To Log Transform Data In SPSS ... Data Transformation for Skewed Variables.
Preferring Box-Cox transformation instead of log transformation to
14 avr. 2022 Background: While dealing with skewed outcome researchers often use log-transformation to convert the data.
Assessing normality
A logarithmic transformation may be useful in normalizing distributions that have more severe positive skew than a square-root transformation. Such distribution
AssessingNormality
Exploring Data: The Beast of Bias
haven't told SPSS which variables we want to plot. Log transformation (log(Xi)): Taking the logarithm of a set of numbers squashes the right tail of the ...
exploringdata
Improving your data transformations: Applying the Box-Cox
12 oct. 2010 traditional transformations (e.g. square root
Data Analysis Toolkit #3: Tools for Transforming Data Page 1
data are right-skewed (clustered at lower values) move down the ladder of powers (that is try square root
Toolkit
Data Transformation Handout
Use this transformation method. Moderately positive skewness. Square-Root. NEWX = SQRT(X). Substantially positive skewness. Logarithmic (Log 10).
data transformation handout
Logarithms and log-transformations
transform skewed data to make the distribution of the data more symmetrical and this helps LN(x) in SPSS and EXCEL and either ln(x) or log(x) in STATA.
logarithmsandlogtransformations
Statistical Approaches for Highly Skewed Data: Evaluating Relations
20 fév. 2020 be transformed using natural log or inverse transformation approaches. Despite these efforts NSSI data often remain highly skewed after ...
Gonzalez Blanks Bridgewater and Yates Statistical Approaches for Highly Skewed Data
Log-transformation and its implications for data analysis
15 mai 2014 software packages including SAS Splus and SPSS. ... the log-transformed data yi is clearly left-skewed. In fact
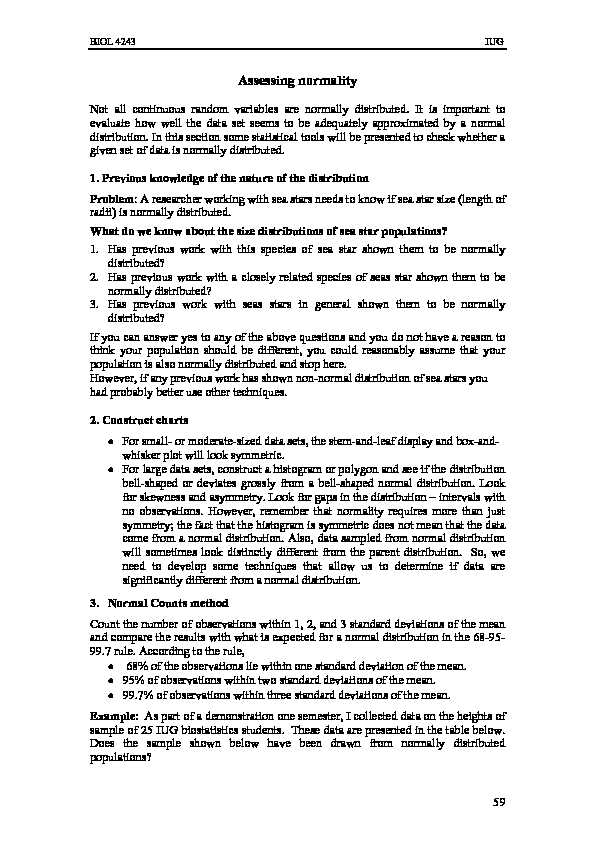
BIOL 4243 IUG
59Assessing normality
Not all continuous random variables are normally distributed. It is important to evaluate how well the data set seems to be adequately approximated by a normal distribution. In this section some statistical tools will be presented to check whether a given set of data is normally distributed.1. Previous knowledge of the nature of the distribution
Problem: A researcher working with sea stars needs to know if sea star size (length of radii) is normally distributed. What do we know about the size distributions of sea star populations?1. Has previous work with this species of sea star shown them to be normally
distributed?2. Has previous work with a closely related species of seas star shown them to be
normally distributed?3. Has previous work with seas stars in general shown them to be normally
distributed? If you can answer yes to any of the above questions and you do not have a reason to think your population should be different, you could reasonably assume that your population is also normally distributed and stop here. However, if any previous work has shown non-normal distribution of sea stars you had probably better use other techniques.2. Construct charts
For small- or moderate-sized data sets, the stem-and-leaf display and box-and- whisker plot will look symmetric. For large data sets, construct a histogram or polygon and see if the distribution bell-shaped or deviates grossly from a bell-shaped normal distribution. Look for skewness and asymmetry. Look for gaps in the distribution - intervals with no observations. However, remember that normality requires more than just symmetry; the fact that the histogram is symmetric does not mean that the data come from a normal distribution. Also, data sampled from normal distribution will sometimes look distinctly different from the parent distribution. So, we need to develop some techniques that allow us to determine if data are significantly different from a normal distribution.3. Normal Counts method
Count the number of observations within 1, 2, and 3 standard deviations of the mean and compare the results with what is expected for a normal distribution in the 68-95-99.7 rule. According to the rule,
68% of the observations lie within one standard deviation of the mean.
95% of observations within two standard deviations of the mean.
99.7% of observations within three standard deviations of the mean.
Example: As part of a demonstration one semester, I collected data on the heights of sample of 25 IUG biostatistics students. These data are presented in the table below. Does the sample shown below have been drawn from normally distributed populations?BIOL 4243 IUG
60Table. Heights, in inches, of 25 IUG biostatistics students.
71.0 69.0 70.0 72.5 73.0
70.0 71.5 70.5 72.0 71.0
68.5 69.0 69.0 68.5 74.0
67.0 69.0 71.5 66.0 70.0
68.5 74.0 74.5 74.0
Solution:
For normal Counts method, determine the following
Heights, in
inchesFrequency
66 167 1
68.5
3 69 4
70 3
70.5 1
71 2Total = 17
71.5 2
72 172.5 1
73 174 3
74.5 1
Total 24
x = 70.6; s = 2.3 xs is 72.9 to 68.3.17 out of the 24 observations i.e. 17/24 = 0.70 = 70% fall within
xs, i.e. between72.9 and 68.3, which is approximately equal to 68%.There is no reason to doubt that
the sample is drawn from a normal population.4. Compute descriptive summary measures
a. The mean, median and mode will have similar values. b. The interquartile range approximately equal to 1.33 s. c. The range approximately equal 6 s.5. Evaluate normal probability plot
If the data come from a normal or approximately normal distribution, the plotted points will fall approximately along a straight line (a 45 degree line). However, if your sample departs from normality, the points on the graph will deviate from that line. If they trail off from a straight-line pack in a curve at the "top" end, observed values bigger than expected, that's right skewed (see below).If the observed values trail off at the bottom end, that's left skewed. Realize that it is important note that any worthwhile computer statistics package will construct these graphs for you (see below).BIOL 4243 IUG
616. Measure of Skewness and Kurtosis
Skewness: The normal distribution is symmetrical. Asymmetrical distributions are sometimes called skewed. Skewness is calculated as follows: 3 1 3 (-1)(-2) n i i nxx skewnesssn n where x is the mean, s is the standard deviation, and n is the number of data points A perfectly normal distribution will have a skewness statistic of zero. If this statistic departs significantly from 0, then we lose confidence that our sample comes from a normally distributed population. If it is negative, then the distribution is skewed to the left or negatively skewed distribution. If it is positive, then the distribution is skewed right or positively skewed distribution.Negatively skewed distribution
or Skewed to the leftSkewness <0 Normal distribution
Symmetrical
Skewness = 0 Positively skewed distribution
or Skewed to the rightSkewness > 0
Kurtosis: A "bell curve" will also depart from normality if the "tails" fail to fall off at the proper rate. If they decrease too fast, the distribution ends up too "peaked." If they don't decrease fast enough, the distribution is too flat in the middle and too fat in the tails. One statistic commonly used to measure kurtosis is typically calculated using the formula, 42(1) 3(1) ( 1)( 2)( 3) ( 2)( 3) i xxnn nkurtosisnnn s nn where x is the mean, s is the standard deviation, and n is the number of data points A perfectly normal distribution will also have a kurtosis statistic of zero. If kurtosis is significantly less than zero, then our distribution is 'flat', it is said to be platykurtic. If kurtosis is significantly greater than 0, the distribution is 'pointed' or peaked, it is called leptokurtic.
Platykurtic distribution
Low degree of peakedness
Kurtosis <0 Normal distribution
Mesokurtic distribution
Kurtosis = 0 Leptokurtic distribution
High degree of peakedness
Kurtosis > 0
BIOL 4243 IUG
62Youwon't have you calculate it by hand. The calculation itself is sensitive to rounding errors because they are raised to the third and fourth powers.
Using SPSS to Evaluate Data for Normality
Before the advent of good computers and statistical programs, users could be forgiven for trying to avoid any surplus calculations. Now that both are available and much easier to use, tests for normality should always be carried out as a best practice in statistics. SPSS offers a variety of methods for evaluating normality.Normal probability plot (P-P plot)
The P-P plot graphs the expected cumulative probability against the observed cumulative probability.1. Open the SPSS file containing your data.
2. From the main menu, select
Graph and then P-P... From the list of available
variables, move the variables you wish to analyze to the variable window. If you select multiple variables then SPSS will create separate plots for each.3. In the box for
Test Distribution be sure that the pop-up menu is set for a Normal distribution. In addition, be sure that theEstimate from data box is checked.
4. In the box for
Proportion Estimation Formula, select the radio button for theRankit method.
5. Finally, in the
Ranks Assigned to Ties box, select the radio button for High.6. Click on
OK to obtain the plot and complete your analysis
BIOL 4243 IUG
59Assessing normality
Not all continuous random variables are normally distributed. It is important to evaluate how well the data set seems to be adequately approximated by a normal distribution. In this section some statistical tools will be presented to check whether a given set of data is normally distributed.1. Previous knowledge of the nature of the distribution
Problem: A researcher working with sea stars needs to know if sea star size (length of radii) is normally distributed. What do we know about the size distributions of sea star populations?1. Has previous work with this species of sea star shown them to be normally
distributed?2. Has previous work with a closely related species of seas star shown them to be
normally distributed?3. Has previous work with seas stars in general shown them to be normally
distributed? If you can answer yes to any of the above questions and you do not have a reason to think your population should be different, you could reasonably assume that your population is also normally distributed and stop here. However, if any previous work has shown non-normal distribution of sea stars you had probably better use other techniques.2. Construct charts
For small- or moderate-sized data sets, the stem-and-leaf display and box-and- whisker plot will look symmetric. For large data sets, construct a histogram or polygon and see if the distribution bell-shaped or deviates grossly from a bell-shaped normal distribution. Look for skewness and asymmetry. Look for gaps in the distribution - intervals with no observations. However, remember that normality requires more than just symmetry; the fact that the histogram is symmetric does not mean that the data come from a normal distribution. Also, data sampled from normal distribution will sometimes look distinctly different from the parent distribution. So, we need to develop some techniques that allow us to determine if data are significantly different from a normal distribution.3. Normal Counts method
Count the number of observations within 1, 2, and 3 standard deviations of the mean and compare the results with what is expected for a normal distribution in the 68-95-99.7 rule. According to the rule,
68% of the observations lie within one standard deviation of the mean.
95% of observations within two standard deviations of the mean.
99.7% of observations within three standard deviations of the mean.
Example: As part of a demonstration one semester, I collected data on the heights of sample of 25 IUG biostatistics students. These data are presented in the table below. Does the sample shown below have been drawn from normally distributed populations?BIOL 4243 IUG
60Table. Heights, in inches, of 25 IUG biostatistics students.
71.0 69.0 70.0 72.5 73.0
70.0 71.5 70.5 72.0 71.0
68.5 69.0 69.0 68.5 74.0
67.0 69.0 71.5 66.0 70.0
68.5 74.0 74.5 74.0
Solution:
For normal Counts method, determine the following
Heights, in
inchesFrequency
66 167 1
68.5
3 69 4
70 3
70.5 1
71 2Total = 17
71.5 2
72 172.5 1
73 174 3
74.5 1
Total 24
x = 70.6; s = 2.3 xs is 72.9 to 68.3.17 out of the 24 observations i.e. 17/24 = 0.70 = 70% fall within
xs, i.e. between72.9 and 68.3, which is approximately equal to 68%.There is no reason to doubt that
the sample is drawn from a normal population.4. Compute descriptive summary measures
a. The mean, median and mode will have similar values. b. The interquartile range approximately equal to 1.33 s. c. The range approximately equal 6 s.5. Evaluate normal probability plot
If the data come from a normal or approximately normal distribution, the plotted points will fall approximately along a straight line (a 45 degree line). However, if your sample departs from normality, the points on the graph will deviate from that line. If they trail off from a straight-line pack in a curve at the "top" end, observed values bigger than expected, that's right skewed (see below).If the observed values trail off at the bottom end, that's left skewed. Realize that it is important note that any worthwhile computer statistics package will construct these graphs for you (see below).BIOL 4243 IUG
616. Measure of Skewness and Kurtosis
Skewness: The normal distribution is symmetrical. Asymmetrical distributions are sometimes called skewed. Skewness is calculated as follows: 3 1 3 (-1)(-2) n i i nxx skewnesssn n where x is the mean, s is the standard deviation, and n is the number of data points A perfectly normal distribution will have a skewness statistic of zero. If this statistic departs significantly from 0, then we lose confidence that our sample comes from a normally distributed population. If it is negative, then the distribution is skewed to the left or negatively skewed distribution. If it is positive, then the distribution is skewed right or positively skewed distribution.Negatively skewed distribution
or Skewed to the leftSkewness <0 Normal distribution
Symmetrical
Skewness = 0 Positively skewed distribution
or Skewed to the rightSkewness > 0
Kurtosis: A "bell curve" will also depart from normality if the "tails" fail to fall off at the proper rate. If they decrease too fast, the distribution ends up too "peaked." If they don't decrease fast enough, the distribution is too flat in the middle and too fat in the tails. One statistic commonly used to measure kurtosis is typically calculated using the formula, 42(1) 3(1) ( 1)( 2)( 3) ( 2)( 3) i xxnn nkurtosisnnn s nn where x is the mean, s is the standard deviation, and n is the number of data points A perfectly normal distribution will also have a kurtosis statistic of zero. If kurtosis is significantly less than zero, then our distribution is 'flat', it is said to be platykurtic. If kurtosis is significantly greater than 0, the distribution is 'pointed' or peaked, it is called leptokurtic.
Platykurtic distribution
Low degree of peakedness
Kurtosis <0 Normal distribution
Mesokurtic distribution
Kurtosis = 0 Leptokurtic distribution
High degree of peakedness
Kurtosis > 0
BIOL 4243 IUG
62Youwon't have you calculate it by hand. The calculation itself is sensitive to rounding errors because they are raised to the third and fourth powers.