Taylor Expansion and Derivative Formulas for Matrix Logarithms
I give the derivation of formulas for the Taylor expansion and derivative of a matrix logarithm. log(x + y) - log(x) - log(x + y + U) + log(x + U) =.
matrixlog tex( )
“Covariates impacts in compositional models and simplicial
2 dic 2019 logarithm is on the right hand side of the regression equation) or symmetrically the partial derivative of E(log(Y )) with respect to X in ...
wp tse
“Covariates impacts in compositional models and simplicial
2 dic 2019 logarithm is on the right hand side of the regression equation) or symmetrically the partial derivative of E(log(Y )) with respect to X in ...
wp tse
DIFFERENTIAL EQUATIONS
Now substituting x = 1 in the above
leep
Impact of covariates in compositional models and simplicial derivatives
25 mar 2021 with respect to log(X) (if the logarithm is on the right hand side of the regression equation) or symmetrically the partial derivative of ...
Impact of Covariates in Compositional Models and S
On a Linear Differential Equation of the Second Order
zero and consequently the fundamental integrals of the equation in the domain of x = O are Yi = ii (x)
CONTINUITY AND DIFFERENTIABILITY
Then we say logarithm of a to base b is x if bx=a log. = x b. 6. logb b = 1 and logb 1 = 0. (iv) The derivative of ex w.r.t.
leep
Differential Equations Assignment #1: answers.
One quickly checks that x(t) = log(t) · et2 is a solution of the equation and satisfies the initial condition; it is even unique as such by the Cauchy-
A S
Dimensions of Logarithmic Quantities
note that eq 1 may be written in a differential form: (x + d log (x) = log (x + dje) - tion multiplying each equation by the stoichiometric num-.
A REFINEMENT OF SELBERG'S ASYMPTOTIC EQUATION
ψ(x) - Σ Λ{n) - Σ log p R{x)= ψ(x) - x we have the two equivalent forms of Selberg's asymptotic equation. (1). R(x)log x + J*Λ(-
) df(t) = O(x)
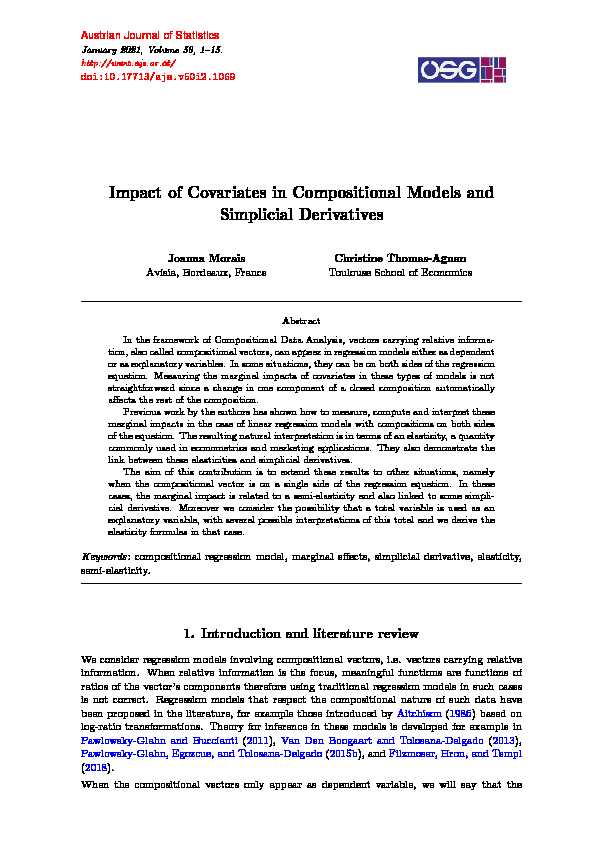
Austrian Journal of Statistics
January 2021, Volume 50, 1{15.
http://www.ajs.or.at/ doi:10.17713/ajs.v50i2.1069Impact of Covariates in Compositional Models andSimplicial Derivatives
Joanna Morais
Avisia, Bordeaux, France
Christine Thomas-Agnan
Toulouse School of EconomicsAbstract
In the framework of Compositional Data Analysis, vectors carrying relative informa- tion, also called compositional vectors, can appear in regression models either as dependent or as explanatory variables. In some situations, they can be on both sides of the regression equation. Measuring the marginal impacts of covariates in these types of models is not straightforward since a change in one component of a closed composition automatically aects the rest of the composition. Previous work by the authors has shown how to measure, compute and interpret these marginal impacts in the case of linear regression models with compositions on both sides of the equation. The resulting natural interpretation is in terms of an elasticity, a quantity commonly used in econometrics and marketing applications. They also demonstrate the link between these elasticities and simplicial derivatives. The aim of this contribution is to extend these results to other situations, namely when the compositional vector is on a single side of the regression equation. In these cases, the marginal impact is related to a semi-elasticity and also linked to some simpli- cial derivative. Moreover we consider the possibility that a total variable is used as an explanatory variable, with several possible interpretations of this total and we derive the elasticity formulas in that case. Keywords: compositional regression model, marginal eects, simplicial derivative, elasticity, semi-elasticity.1. Introduction and literature review We consider regression models involving compositional vectors, i.e. vectors carrying relative information. When relative information is the focus, meaningful functions are functions of ratios of the vector's components therefore using traditional regression models in such cases is not correct. Regression models that respect the compositional nature of such data have been proposed in the literature, for example those introduced byAi tchison
1986) based on log-ratio transformations. Theory for inference in these models is developed for example in
Pawlowsky-Glahn and Buccianti
2011V anD enBo ogaartan dT olosana-Delgado
2013Pawlowsky-Glahn, Egozcue, and Tolosana-Delgado
2015b),and
Fi lzmoser,Hr on,an dT empl
2018When the compositional vectors only appear as dependent variable, we will say that the
2Impacts of Covariates
model is of the `Y-compositional' type (see e.g.E gozcue,D aunis-I-Estadella,P awlowsky-
Glahn, Hron, and Filzmoser
2012)). When they only appear as explanatory variables, we will say that the model is of the `X-compositional' type (see e.g.
Hron ,Fi lzmoser,and
Thompson
2012)). Finally, when they appear on both sides, we will say that the model is of the `YX-compositional' type, see e.g.
K ynclova,Fi lzmoser,and Hr on
2015Ch en,Zh ang,
and Li 2017M orais,T homas-Agnan,an dS imioni
2018a) and
M orais,Th omas-Agnan,
and Simioni 2018b). A simplied version of the YX-compositional type is presented in AJS
Austrian Journal of Statistics
January 2021, Volume 50, 1{15.
http://www.ajs.or.at/ doi:10.17713/ajs.v50i2.1069Impact of Covariates in Compositional Models andSimplicial Derivatives
Joanna Morais
Avisia, Bordeaux, France
Christine Thomas-Agnan
Toulouse School of EconomicsAbstract
In the framework of Compositional Data Analysis, vectors carrying relative informa- tion, also called compositional vectors, can appear in regression models either as dependent or as explanatory variables. In some situations, they can be on both sides of the regression equation. Measuring the marginal impacts of covariates in these types of models is not straightforward since a change in one component of a closed composition automatically aects the rest of the composition. Previous work by the authors has shown how to measure, compute and interpret these marginal impacts in the case of linear regression models with compositions on both sides of the equation. The resulting natural interpretation is in terms of an elasticity, a quantity commonly used in econometrics and marketing applications. They also demonstrate the link between these elasticities and simplicial derivatives. The aim of this contribution is to extend these results to other situations, namely when the compositional vector is on a single side of the regression equation. In these cases, the marginal impact is related to a semi-elasticity and also linked to some simpli- cial derivative. Moreover we consider the possibility that a total variable is used as an explanatory variable, with several possible interpretations of this total and we derive the elasticity formulas in that case. Keywords: compositional regression model, marginal eects, simplicial derivative, elasticity, semi-elasticity.1. Introduction and literature review We consider regression models involving compositional vectors, i.e. vectors carrying relative information. When relative information is the focus, meaningful functions are functions of ratios of the vector's components therefore using traditional regression models in such cases is not correct. Regression models that respect the compositional nature of such data have been proposed in the literature, for example those introduced byAi tchison
1986) based on log-ratio transformations. Theory for inference in these models is developed for example in
Pawlowsky-Glahn and Buccianti
2011V anD enBo ogaartan dT olosana-Delgado
2013Pawlowsky-Glahn, Egozcue, and Tolosana-Delgado
2015b),and
Fi lzmoser,Hr on,an dT empl
2018When the compositional vectors only appear as dependent variable, we will say that the
2Impacts of Covariates
model is of the `Y-compositional' type (see e.g.E gozcue,D aunis-I-Estadella,P awlowsky-
Glahn, Hron, and Filzmoser
2012)). When they only appear as explanatory variables, we will say that the model is of the `X-compositional' type (see e.g.
Hron ,Fi lzmoser,and
Thompson
2012)). Finally, when they appear on both sides, we will say that the model is of the `YX-compositional' type, see e.g.
K ynclova,Fi lzmoser,and Hr on
2015Ch en,Zh ang,
and Li 2017M orais,T homas-Agnan,an dS imioni
2018a) and
M orais,Th omas-Agnan,
and Simioni 2018b). A simplied version of the YX-compositional type is presented in
- log x differentiation formula