Covariance and Correlation
28 Jul 2017 The reverse is not true in general: if the covariance of two random variables is 0 they can still be dependent! Page 2. –2–. Properties of ...
covariance
Scatterplots and Correlation
Measuring Linear Association: Correlation Calculate and interpret correlation. ... and motivation scores in this example range from 0 to 100.
scatterplots and correlation notes
Reminder No. 1: Uncorrelated vs. Independent
27 Feb 2013 If ρ(XY) = 0
uncorrelated vs independent
Pearson's correlation
We can categorise the type of correlation by considering as one variable increases The first three represent the “extreme” correlation values of -1 0.
pearsons
New Automatic Search Tool for Impossible Differentials and Zero
Abstract. Impossible differential and zero-correlation linear cryptanalysis are two of the most powerful cryptanalysis methods in the field of symmetric key
Links among Impossible Differential Integral and Zero Correlation
Secondly by establishing some boolean equations
The Bivariate Normal Distribution
Zero Correlation Implies Independence. If two random variables X and Y are jointly normal and are uncorrelated then they are independent.
Bivariate Normal
Zero Correlation Independence
https://www.tandfonline.com/doi/pdf/10.1080/00031305.1986.10475412
Correlation coefficient and p-values: what they are and why you
The p-value is a number between 0 and 1 representing the probability that this data would have arisen if the null hypothesis were true. In medical trials the
p values
1.10.5 Covariance and Correlation
2. If random variables X1 and X2 are independent then cov(X1X2)=0. 3. var(aX1 + bX2) =
MS NotesWeek
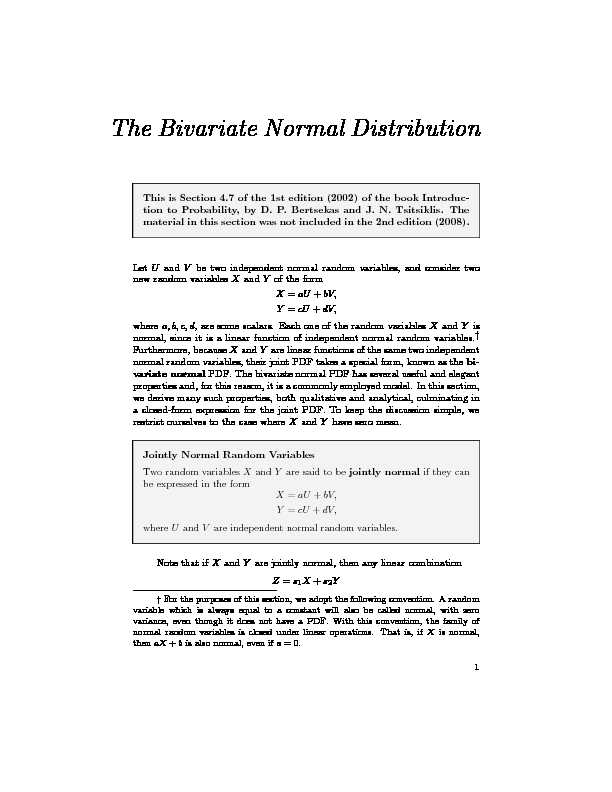
The Bivariate Normal Distribution
This is Section 4.7 of the 1st edition (2002) of the book Introduc- tion to Probability, by D. P. Bertsekas and J. N. Tsitsiklis. The material in this section was not included in the 2nd edition (2008). LetUandVbe two independent normal random variables, and consider two new random variablesXandYof the formX=aU+bV,
Y=cU+dV,
wherea,b,c,d, are some scalars. Each one of the random variablesXandYis normal, since it is a linear function of independent normal random variables. Furthermore, becauseXandYare linear functions of the same two independent normal random variables, their joint PDF takes a special form, known as thebi- variate normalPDF. The bivariate normal PDF has several useful and elegant properties and, for this reason, it is a commonly employed model. In this section, we derive many such properties, both qualitative and analytical, culminating in a closed-form expression for the joint PDF. To keep the discussion simple, we restrict ourselves to the case whereXandYhave zero mean.Jointly Normal Random Variables
Two random variablesXandYare said to bejointly normalif they can beexpressedintheformX=aU+bV,
Y=cU+dV,
whereUandVare independent normal random variables. Note that ifXandYare jointly normal, then any linear combination Z=s 1 X+s 2 Y For the purposes of this section, we adopt the following convention. A random variable which is always equal to a constant will also be called normal, with zero variance, even though it does not have a PDF. With this convention, the family of normal random variables is closed under linear operations. That is, ifXis normal, thenaX+bis also normal, even ifa=0. 12The Bivariate Normal Distribution
has a normal distribution. The reason is that if we haveX=aU+bVand Y=cU+dVfor some independent normal random variablesUandV,then Z=s 1 (aU+bV)+s 2 (cU+dV)=(as 1 +cs 2 )U+(bs 1 +ds 2 )V. Thus,Zis the sum of the independent normal random variables (as 1 +cs 2 )U and (bs 1 +ds 2 )V, and is therefore normal. A very important property of jointly normal random variables, and which will be the starting point for our development, is that zero correlation implies independence.Zero Correlation Implies Independence
If two random variablesXandYare jointly normal and are uncorrelated, then they are independent. This property can be verified using multivariate transforms, as follows. Suppose thatUandVare independent zero-mean normal random variables, and thatX=aU+bVandY=cU+dV,sothatXandYare jointly normal. We assume thatXandYare uncorrelated, and we wish to show that they are independent. Our first step is to derive a formula for the multivariate transform M X,Y (s 1 ,s 2 ) associated withXandY. Recall that ifZis a zero-mean normal random variable with varianceσ 2Z , the associated transform is E[e sZ ]=M Z (s)=e 2Z s 2 /2 which implies that E[e Z ]=M Z (1) =e 2Z /2Let us fix some scalarss
1 ,s 2 ,andletZ=s 1 X+s 2Y. The random variableZ
is normal, by our earlier discussion, with variance 2Z =s 212X +s 22
2Y This leads to the following formula for the multivariate transform associated with the uncorrelated pairXandY: M X,Y (s 1 ,s 2 )=E[e s 1 X+s 2 Y =E[e Z =e (s 21
2X +s 22
2Y )/2. Let nowXandYbeindependentzero-mean normal random variables with the same variancesσ 2X andσ 2Y asXandY, respectively. SinceXandYare independent, they are also uncorrelated, and the preceding argument yields M X,Y (s 1 ,s 2 )=e (s 21
2X +s 22
2Y )/2.
The Bivariate Normal Distribution3
Thus, the two pairs of random variables (X,Y)and(X,Y) are associated with the same multivariate transform. Since the multivariate transform completely determines the joint PDF, it follows that the pair (X,Y) has the same jointPDF as the pair (
X,Y). SinceXandYare independent,XandYmust also
be independent, which establishes our claim.The Conditional Distribution ofXGivenY
We now turn to the problem of estimatingXgiven the value ofY.Toavoid uninteresting degenerate cases, we assume that bothXandYhave positive variance. Let us defineX=ρσ
X YY,X=X-X,
whereρ=E[XY]
X Y is the correlation coefficient ofXandY.SinceXandYare linear combinations of independent normal random variablesUandV, it follows thatYandXareThe Bivariate Normal Distribution
This is Section 4.7 of the 1st edition (2002) of the book Introduc- tion to Probability, by D. P. Bertsekas and J. N. Tsitsiklis. The material in this section was not included in the 2nd edition (2008). LetUandVbe two independent normal random variables, and consider two new random variablesXandYof the formX=aU+bV,
Y=cU+dV,
wherea,b,c,d, are some scalars. Each one of the random variablesXandYis normal, since it is a linear function of independent normal random variables. Furthermore, becauseXandYare linear functions of the same two independent normal random variables, their joint PDF takes a special form, known as thebi- variate normalPDF. The bivariate normal PDF has several useful and elegant properties and, for this reason, it is a commonly employed model. In this section, we derive many such properties, both qualitative and analytical, culminating in a closed-form expression for the joint PDF. To keep the discussion simple, we restrict ourselves to the case whereXandYhave zero mean.Jointly Normal Random Variables
Two random variablesXandYare said to bejointly normalif they can beexpressedintheformX=aU+bV,
Y=cU+dV,
whereUandVare independent normal random variables. Note that ifXandYare jointly normal, then any linear combination Z=s 1 X+s 2 Y For the purposes of this section, we adopt the following convention. A random variable which is always equal to a constant will also be called normal, with zero variance, even though it does not have a PDF. With this convention, the family of normal random variables is closed under linear operations. That is, ifXis normal, thenaX+bis also normal, even ifa=0. 12The Bivariate Normal Distribution
has a normal distribution. The reason is that if we haveX=aU+bVand Y=cU+dVfor some independent normal random variablesUandV,then Z=s 1 (aU+bV)+s 2 (cU+dV)=(as 1 +cs 2 )U+(bs 1 +ds 2 )V. Thus,Zis the sum of the independent normal random variables (as 1 +cs 2 )U and (bs 1 +ds 2 )V, and is therefore normal. A very important property of jointly normal random variables, and which will be the starting point for our development, is that zero correlation implies independence.Zero Correlation Implies Independence
If two random variablesXandYare jointly normal and are uncorrelated, then they are independent. This property can be verified using multivariate transforms, as follows. Suppose thatUandVare independent zero-mean normal random variables, and thatX=aU+bVandY=cU+dV,sothatXandYare jointly normal. We assume thatXandYare uncorrelated, and we wish to show that they are independent. Our first step is to derive a formula for the multivariate transform M X,Y (s 1 ,s 2 ) associated withXandY. Recall that ifZis a zero-mean normal random variable with varianceσ 2Z , the associated transform is E[e sZ ]=M Z (s)=e 2Z s 2 /2 which implies that E[e Z ]=M Z (1) =e 2Z /2Let us fix some scalarss
1 ,s 2 ,andletZ=s 1 X+s 2Y. The random variableZ
is normal, by our earlier discussion, with variance 2Z =s 212X +s 22
2Y This leads to the following formula for the multivariate transform associated with the uncorrelated pairXandY: M X,Y (s 1 ,s 2 )=E[e s 1 X+s 2 Y =E[e Z =e (s 21
2X +s 22
2Y )/2. Let nowXandYbeindependentzero-mean normal random variables with the same variancesσ 2X andσ 2Y asXandY, respectively. SinceXandYare independent, they are also uncorrelated, and the preceding argument yields M X,Y (s 1 ,s 2 )=e (s 21
2X +s 22
2Y )/2.