Course designed for the biomedical researcher Topics include: descriptive statistics, hypothesis testing, estimation, confidence
Biostatistics and Medical Informatics 1 BIOSTATISTICS AND MEDICAL INFORMATICS DEGREES/MAJORS, DOCTORAL MINORS, GRADUATE/PROFESSIONAL CERTIFICATES
Public Health Concentration Courses: Biostatistics Public Health Informatics Option - Approved courses for the 14-16 catalog
Core Mission • To serve as a source of expertise in epidemiology, biostatistics, and informatics specific to cancer and aging research, to promote the use
Learn from supportive, accessible faculty in biostatistics, informatics, genetics, medicine and public health • Grow as an integral member of a research
informatics research, a literature review of recent articles in two high-impact factor biomedical level of biostatistical competence be demonstrated
disease, health informatics and data analytics, big data, data capture, management analysis for large clinical trial studies Graduate Degrees
IGPI:3510 Biostatistics 3 s h Statistical concepts and methods for the biological sciences; descriptive statistics, elementary probability,
27 mar 2019 · Biostatistics and Health Informatics understand the major issues related to applying informatics techniques to transforming medical data
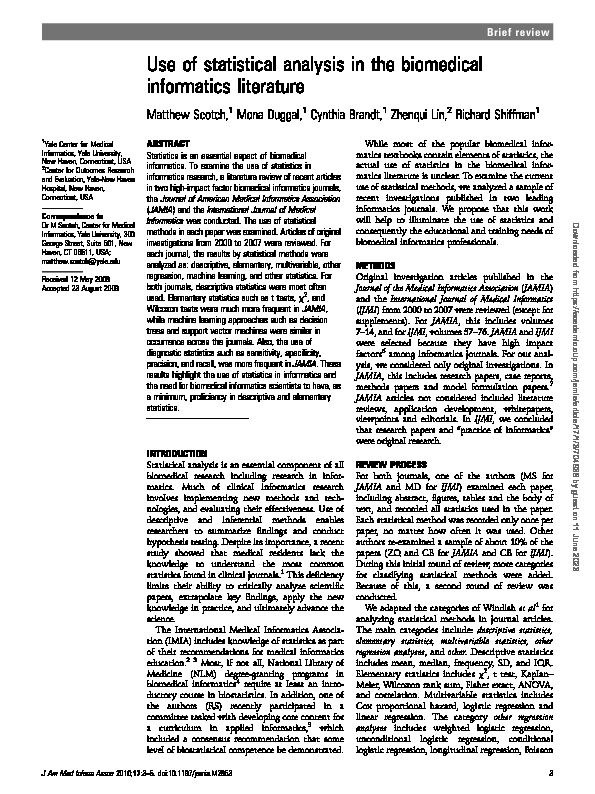
33426_617_1_3.pdf
Use of statistical analysis in the biomedical
informatics literature
Matthew Scotch,
1
Mona Duggal,
1
Cynthia Brandt,
1
Zhenqui Lin,
2
Richard Shiffman
1
ABSTRACT
Statistics is an essential aspect of biomedical
informatics. To examine the use of statistics in informatics research, a literature review of recent articles in two high-impact factor biomedical informatics journals, theJournal of American Medical Informatics Association (
JAMIA) and theInternational Journal of Medical
Informaticswas conducted. The use of statistical
methods in each paper was examined. Articles of original investigations from 2000 to 2007 were reviewed. For each journal, the results by statistical methods were analyzed as: descriptive, elementary, multivariable, other regression, machine learning, and other statistics. For both journals, descriptive statistics were most often used. Elementary statistics such as t tests,x2 , and
Wilcoxon tests were much more frequent inJAMIA,
while machine learning approaches such as decision trees and support vector machines were similar in occurrence across the journals. Also, the use of diagnostic statistics such as sensitivity, specificity, precision, and recall, was more frequent inJAMIA. These results highlight the use of statistics in informatics and the need for biomedical informatics scientists to have, as a minimum, proficiency in descriptive and elementary statistics.
INTRODUCTION
Statistical analysis is an essential component of all biomedical research including research in infor- matics. Much of clinical informatics research involves implementing new methods and tech- nologies, and evaluating their effectiveness. Use of descriptive and inferential methods enables researchers to summarizefindings and conduct hypothesis testing. Despite its importance, a recent study showed that medical residents lack the knowledge to understand the most common statistics found in clinical journals.1
This deficiency
limits their ability to critically analyze scientific papers, extrapolate keyfindings, apply the new knowledge in practice, and ultimately advance the science.
The International Medical Informatics Associa-
tion (IMIA) includes knowledge of statistics as part of their recommendations for medical informatics education. 23
Most, if not all, National Library of
Medicine (NLM) degree-granting programs in
biomedical informatics 4 require at least an intro- ductory course in biostatistics. In addition, one of the authors (RS) recently participated in a committee tasked with developing core content for a curriculum in applied informatics,5 which included a consensus recommendation that some level of biostatistical competence be demonstrated.While most of the popular biomedical infor- matics textbooks contain elements of statistics, the actual use of statistics in the biomedical infor- matics literature is unclear. To examine the current use of statistical methods, we analyzed a sample of recent investigations published in two leading informatics journals. We propose that this work will help to illuminate the use of statistics and consequently the educational and training needs of biomedical informatics professionals.
METHODS
Original investigation articles published in the
Journal of the Medical Informatics Association(JAMIA) and theInternational Journal of Medical Informatics (IJMI) from 2000 to 2007 were reviewed (except for supplements). ForJAMIA, this includes volumes
7-14, and forIJMI, volumes 57-76.JAMIAandIJMI
were selected because they have high impact factors6 among informatics journals. For our anal- ysis, we considered only original investigations. In JAMIA , this includes research papers, case reports, methods papers and model formulation papers. 7
JAMIAarticles not considered included literature
reviews, application development, whitepapers, viewpoints and editorials. InIJMI, we concluded that research papers and"practice of informatics" were original research.REVIEW PROCESS
For both journals, one of the authors (MS for
JAMIAand MD forIJMI) examined each paper,
including abstract,figures, tables and the body of text, and recorded all statistics used in the paper. Each statistical method was recorded only once per paper, no matter how often it was used. Other authors re-examined a sample of about 10% of the papers (ZQ and CB forJAMIAand CB forIJMI). During this initial round of review, more categories for classifying statistical methods were added.
Because of this, a second round of review was
conducted.
We adapted the categories of Windishet al
1 for analyzing statistical methods in journal articles. The main categories include:descriptive statistics, elementary statistics, multivariable statistics, other regression analyses,andother. Descriptive statistics includes mean, median, frequency, SD, and IQR.
Elementary statistics includesx2
,ttest,Kaplan-
Meier, Wilcoxon rank sum, Fisher exact, ANOVA,
and correlation. Multivariable statistics includes
Cox proportional hazard, logistic regression and
linear regression. The categoryother regression analysesincludes weighted logistic regression, unconditional logistic regression, conditional logistic regression, longitudinal regression, Poisson1
Yale Center for Medical
Informatics, Yale University,
New Haven, Connecticut, USA
2
Center for Outcomes Research
and Evaluation, Yale-New Haven
Hospital, New Haven,
Connecticut, USA
Correspondence to
Dr M Scotch, Center for Medical
Informatics, Yale University, 300
George Street, Suite 501, New
Haven, CT 06511, USA;
matthew.scotch@yale.edu
Received 12 May 2008
Accepted 23 August 2009
J Am Med Inform Assoc2010;17:3-5. doi:10.1197/jamia.M28533
Brief reviewDownloaded from https://academic.oup.com/jamia/article/17/1/3/704938 by guest on 11 June 2023
regression, pooled logistic regression, nonlinear regression, negative binomial regression, and generalized estimating equa- tions. Another category,machine learning and data mining(not included in Windishet al), includes statistical classifiers such as Bayesian networks, decision trees, artificial neural networks and support vector machines. This gro up also includes unsupervised learning methods such as clustering. Finally, the categoryother statisticsincludes mostly classification and diagnostic test analyses such as relative risk/risk ratio, sensitivity/specificity and precision/recall.
RESULTS
From 2000 to 2007, we identified 305JAMIApapers and 532IJMI papers that met our inclusion criterion. For theJAMIAarticles, articles were also strati fied by article type: research papers, case reports, methods papers, and model formulation papers (table 1). IJMIpapers were not stratified because the type of the original investigation was not always indicated by the journal. Table 2 shows the number (and percentage) of articles in which each type of test was applied for each journal. A sample of 10% (31) of theJAMIApapers had an observed agreement of 0.95 (k50.85) among two of the reviewers (MS and CB). A sample of 10% (53) of theIJMIpapers had an observed agreement of 0.90 (k50.63) among two of the reviewers (MD and CB). Descriptive statistics such as mean and SD were by far the most frequently used in both biomedical informatics journals. Elementary statistics including parametric and non-parametric tests were used in 42% of theJAMIAstudies, while only 22% of
theIJMIpapers used these types of statistics. Statistics that areoften used for clinical reasoning and decision-making, such as
sensitivity, specificity, precision and recall, were more frequent in JAMIAthanIJMI. For multivariable statistics including regres- sion,JAMIAhad 12% of these, whileIJMIhad 6%. Finally, data- mining and machine-learning methods such as support vector machines, decision trees, and Bayesian networks were 9% in
JAMIAand 6% inIJMI.
DISCUSSION
Original investigations frequently include statistical analysis. In our results, the use of descriptive and elementary statistics was high. In addition, diagnostic statistics such as sensitivity, specificity, precision, and recall, a popular approach for those original studies using statistics, was frequently used inJAMIA articles. This is not surprising, since much of the informaticsfield revolves around use of computers to assist decision-making (in medicine, public health, etc) as well as evaluation of different methods for retrieving biomedical information. Clinicians are taught methods for reasoning under uncertainty and the use of sensitivity, specificity and other diagnostic statistics. Biomedical informatics trainees without clinical backgrounds are taught these statistics through introductory biomedical informatics coursework and textbooks such asMedical Informatics: Computer
Applications in Health Care
8 andEvaluation Methods in Biomedical
Informatics.
9 Descriptive or elementary knowledge of statistics is needed for informatics research such as decision-support system evaluation, understanding the barriers to Electronic Medical Record imple- mentation, information retrieval, summarization of phyloge- netic analysis, or spatial clustering for outbreak detection. In Table 1Statistical method by type ofJAMIAarticle (2000-2007)
Type of testStudy type, n (%)
Research papers (n5228) Case report (n537) Methods papers (n54) Model formulation (n536)
No statistics22 (10%)12 (32%)0 (0%)21 (58%)
Descriptive statistics177 (78%)23 (62%)3 (75%)13 (36%) Elementary statistics116 (51%)11 (30%)1 (25%)1 (3%) x 2
Analysis51 (22%)5 (14%)0 (0%)0 (0%)
t Test48 (21%)4 (11%)0 (0%)0 (0%)
Kaplan-Meier analysis2 (1%)0 (0%)0 (0%)0 (0%)
Wilcoxon rank sum test24 (11%)0 (0%)0 (0%)0 (0%)
Sign test1 (0%)0 (0%)0 (0%)0 (0%)
Fisher exact test11 (5%)1 (3%)0 (0%)0 (0%)
Analysis of variance36 (16%)0 (0%)0 (0%)1 (3%)
Correlation45 (20%)4 (11%)1 (25%)1 (3%)
Multivariable statistics37 (16%)1 (3%)0 (0%)0 (0%) Multiple logistic regression22 (10%)1 (3%)0 (0%)0 (0%) Multiple linear regression15 (7%)0 (0%)0 (0%)0 (0%) Principal-component analysis4 (2%)0 (0%)0 (0%)0 (0%)
Other regression analyses9 (4%)0 (0%)0 (0%)0 (0%)
Data mining/machine learning24 (11%)1 (3%)1 (25%)2 (6%)
Support vector machines5 (2%)1 (3%)0 (0%)0 (0%)
Bayesian network11 (5%)0 (0%)1 (25%)0 (0%)
Neural networks1 (0%)0 (0%)0 (0%)0 (0%)
Decision trees8 (4%)0 (0%)0 (0%)0 (0%)
Clustering1 (0%)0 (0%)0 (0%)1 (3%)
Hidden Markov Monte Carlo3 (1%)0 (0%)0 (0%)1 (3%)
Other statistics65 (29%)4 (11%)2 (50%)2 (6%)
Relative risk/risk ratio7 (3%)0 (0%)0 (0%)0 (0%)
Sensitivity/specificity, precision/recall 56 (25%)4 (11%)1 (25%)2 (6%)
Fuzzy logic1 (0%)0 (0%)0 (0%)0 (0%)
Latent semantic analysis2 (1%)0 (0%)0 (0%)0 (0%)
Fourier transform/time series2 (1%)0 (0%)1 (25%)0 (0%)
4J Am Med Inform Assoc2010;17:3-5. doi:10.1197/jamia.M2853
Brief reviewDownloaded from https://academic.oup.com/jamia/article/17/1/3/704938 by guest on 11 June 2023
fact, many studies in clinical settings (eg, clinical trials) require even more sophisticated techniques. Knowledge of statistics, therefore, is important not only for those conducting research studies, but also for understanding thefindings in the biomedical informatics literature and scientific presentations. Development of biomedical informatics training requirements and competencies in statistics must be done with the consid- eration that the current statistical methods used in these jour-
nals might not always represent the most appropriate methodsto analyzing data. There is the potential that statistical tests notoften used in thefield would greatly enhance the analysis of
biomedical informatics research. Careful consideration must be done whenfinalizing training requirements and competency guidelines.
LIMITATIONS
Our study focused on articles in theJAMIAand theIJMI. These journals publish articles from all different foci within biomedical informatics. This includes bioinformatics, which is one of the most popular and growing disciplines within thefield.JAMIAis more clinically oriented, and the number of bioinformatics studies is small. Thus, our results likely do not account for the breadth of statistics in these studies which, because of the nature of this discipline, can be more sophisticated.
CONCLUSIONS
As core competencies and credentialing in biomedical infor- matics are developed, scientists in thisfield should have, as a minimum, proficiency in descriptive and elementary statistics. FundingThis project is supported in part by TI5 LM007056 and R01 LM007199 from the National Library of Medicine (NLM).
Competing interestsNone.
Provenance and peer reviewNot commissioned; externally peer reviewed.
REFERENCES
1.Windish DM,Huot SJ, Green ML. Medicine residents' understanding of the
biostatistics and results in the medical literature.JAMA2007;298:1010-22.
2.Johnson SB.A framework for the biomedical informatics curriculum.AMIA Annu
Symp Proc2003;331-5.
3. Recommendations of the International Medical Informatics Association (IMIA) on
education in health and medical informatics.Methods Inf Med2000;39:267-77.
4.NLM. NLM's university-based biomedical informatics research training programs.
2007. http://www.nlm.nih.gov/ep/GrantTrainInstitute.html (accessed 20 Aug 2009).
5. AMIA receives grant from Robert Wood Johnson Foundation to foster the development
of applied clinical informatics as a medical specialty.AMIA News Release2007. http:// www.amia.org/inside/releases/2007/rwjf2007grantannouncement.pdf (accessed 20
Aug 2009).
6.Thomson Corporation. ISI web of knowledge: journal citation reports. 2007. http://
admin-apps.isiknowledge.com/JCR/JCR (accessed 3 Oct 2007).
7.JAMIA. Journal of the American Medical Informatics Association instructions for
authors. 2005. http://www.jamia.org/misc/ifora.shtml (accessed 3 Oct 2007).
8.Shortliffe EH,Cimino JJ. Biomedical informatics: computer applications in health care
and biomedicine. 3rd edn. New York: Springer, 2006.
9.Friedman CP,Wyatt J, Ash J. Evaluation methods in biomedical informatics. 2nd edn.
New York: Springer, 2006.
Table 2Summary of the statistical methods inJAMIAandIJMIarticles (2000-2007)
Type of testJAMIA(n5305)IJMI(n5532)
No (%) No (%)
No statistics55 (18%)189 (36%)
Descriptive statistics216 (71%)328 (62%)
Elementary statistics129 (42%)119 (22%)
x 2
Analysis56 (18%)49 (9%)
t Test52 (17%)44 (8%)
Kaplan-Meier analysis2 (1%)0 (0%)
Wilcoxon rank sum test24 (8%)22 (4%)
Sign test1 (0%)1 (0%)
Fisher exact test12 (4%)9 (2%)
Analysis of variance37 (12%)32 (6%)
Correlation51 (17%)41 (8%)
Multivariable statistics38 (12%)31 (6%)
Multiple logistic regression23 (8%)18 (3%)
Multiple linear regression15 (5%)10 (2%)
Principal-component analysis4 (1%)5 (1%)
Other regression analyses9 (3%)4 (1%)
Data mining/machine learning28 (9%)32 (6%)
Support vector machines6 (2%)4 (1%)
Bayesian network12 (4%)7 (1%)
Neural networks1 (0%)9 (2%)
Decision trees8 (3%)9 (2%)
Clustering2 (1%)4 (1%)
Hidden Markov Monte Carlo4 (1%)5 (1%)
Other statistics73 (24%)68 (13%)
Relative risk/risk ratio7 (2%)3 (1%)
Sensitivity/specificity, precision/recall 63 (21%)62 (12%)
Fuzzy logic1 (0%)2 (0%)
Latent semantic analysis2 (1%)1 (0%)
Fourier transform/time series3 (1%)1 (0%)
Classification format adapted from Windishet al.
1 J Am Med Inform Assoc2010;17:3-5. doi:10.1197/jamia.M28535
Brief reviewDownloaded from https://academic.oup.com/jamia/article/17/1/3/704938 by guest on 11 June 2023