ENFERMEDAD ARTERIAL CORONARIA
En conclusión la ET-1
OBSTETRICIA Y GINECOLOGIA
opiniones o conclusiones expresadas en los artículos son de la exclusiva responsabilidad de los 4 (Cleveland Clinic Incontinence Score) definido.
Laboratorio clínico y oncología: De los aspectos básicos del cáncer
10 jul 2013 iNtRoDuCCióN. En México como en otros países los avances tecnológicos
Explainable Artificial Intelligence: a Systematic Review
13 oct 2020 bols enabling user-driven explanations of how a conclusion is reached” ... [69] David S Watson Jenny Krutzinna
Grounded Knowledge Bases for Scientific Domains
der grant numbers IIS-0811562 CCF-1247088
Lycée polyvalent de Taaone Page 1 sur 25 - Sujet détude : Voyages
- Christophe Colomb et la découverte de l'Amérique. - Le tour du monde de Bougainville. - James Cook et l'exploration du. Pacifique. On montre que les
NGO ASSESSMENT IN TOGO:
EXECUTIVE SUMMARY: NGO ASSESSMENT IN TOGO Colombe NGO- YES ... Christopher Schwabe of MCDI conducted an econometric analysis of the demand for curative ...
Untitled
2 dic 2020 Eduardo Teniza Frias* Christopher Hernández Cortés
SOCCA 30th Annual Meeting and Critical Care Update
5 may 2017 Cleveland Clinic Lerner College of Medicine ... La Colombe Coffee ... Summary: Diagnostic criteria for septic shock include elevation of ...
Salud y cuidados en el envejecimiento. Volumen III
25 abr 2014 Realizando una revisión de artículos llegamos a la conclusión que no hay ... asociadas pulmonares tos crónica
[PDF] le récit des voyages de Christophe Colomb (classe de cinquième)
-?Introduction: origines de Christophe Colomb -?1er §: description du voyage et de ses étapes -?2è §: la rencontre avec les Indiens et ses conséquences
Essai dinterprétation de la signature de Christophe Colomb - Érudit
2 Ses conclusions ne confirment pas en vérité la thèse calvi- nèse et ne justifient donc pas l'apposition de la plaque commemo- rative qui décore une des
[PDF] Histoire de Christophe Colomb
traient pas Christophe Colomb et Las Ca- sas nous ne verrions au milieu des scènes d'horreurs qui ont ensanglanté l'Amérique
[PDF] christophe colomb
CHRISTOPHE COLOMB DEVANT L'IIISTOIRE Fi Dans la iiui t du 11 au '12 octolire 1492 íi deux heures aprés niiiiuit un vendredi Christophc Colornb
DOSSIER : 1492 - Christophe Colomb de la route des Indes au
En 1492 le navigateur génois Christophe Colomb persuadé de pouvoir gagner les Indes par l'ouest traverse l'océan Atlantique pour le compte des souverains
[PDF] Christophe Colomb : drame en sept actes (dix-sept tableaux
Christophe Colomb : drame en sept actes (dix-sept tableaux) / [Gustave Pradelle] 1867 1/ Les contenus accessibles sur le site Gallica sont pour la plupart
Christophe Colomb - Wikipédia
Il est rapidement libéré par les souverains mais perd ses titres de gouverneur et de vice-roi ; lorsqu'il est autorisé à repartir pour un nouveau voyage en
[PDF] Christophe Colomb - Forgotten Books
INTRODUCTION DOCUMENTS MANUSCRITS ET IMPRIMÉS ÉCRITS DE CHRISTOPHE COLOMB HRISTOPHE Colomb a beaucoup écrit Son activité
Christophe Colomb Conclusion - Etudiercom
Introduction et Problématique 2 Portrait de Christophe Colomb ainsi que sa biographie 3 Carte des voyages 4 Gravure du débarquement de Christophe Colomb
Quel est le résultat du voyage de Christophe Colomb ?
Le navigateur et explorateur génois Christophe Colomb est revenu de sa première exploration -découverte du Nouveau Monde (1492-1493)- en héros. Il est reçu triomphalement par les Rois Catholiques -Ferdinand II d'Aragon et son épouse Isabelle de Castille à Barcelone en avril 1493.Quelle est l'importance de la découverte de Christophe Colomb ?
Mais l'arrivée de Christophe Colomb marque le début de la colonisation du continent américain par les Européens. En débarquant dans ce “Nouveau Monde”, les Européens s'approprient les terres des populations locales, que Christophe Colomb appelle les Indiens, puisqu'il croit être en Inde.Quels sont les opinions de Christophe Colomb ?
Il était convaincu de la rotondité de la terre
Si - comme il le pense - la terre est ronde, Christophe Colomb est persuadé qu'il peut atteindre la Chine plus rapidement en prenant le chemin du Ponant.- L'objectif de Christophe Colomb est de récupérer les 39 personnes laissées dans la baie de la Navidad (suite au naufrage de la Santa Maria en décembre 1492 lors du premier voyage) et d'établir une colonie sur l'île d'Hispaniola (aujourd'hui Haïti et République dominicaine).
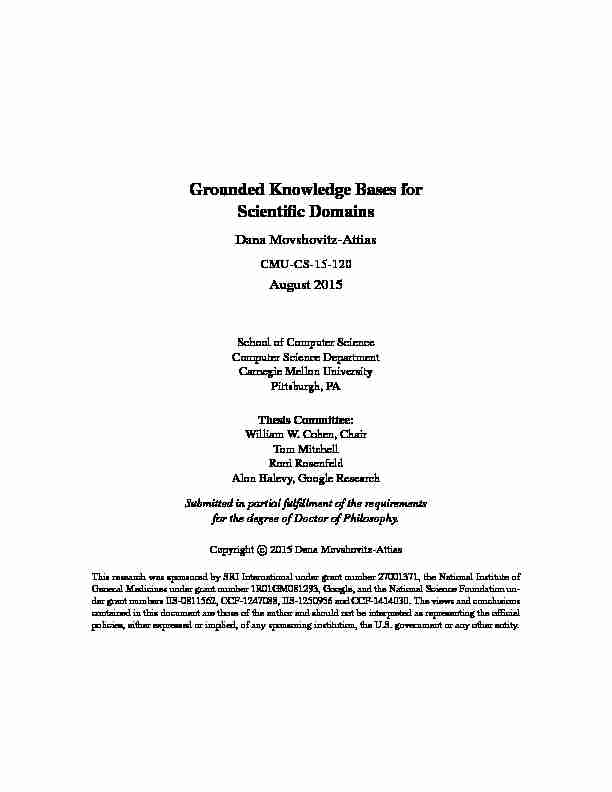
Grounded Knowledge Bases for
Scientific Domains
Dana Movshovitz-Attias
CMU-CS-15-120
August 2015
School of Computer Science
Computer Science Department
Carnegie Mellon University
Pittsburgh, PA
Thesis Committee:
William W. Cohen, Chair
Tom Mitchell
Roni Rosenfeld
Alon Halevy, Google Research
Submitted in partial fulfillment of the requirements for the degree of Doctor of Philosophy.Copyright
c2015 Dana Movshovitz-Attias
This research was sponsored by SRI International under grant number 27001371, the National Institute of
General Medicines under grant number 1R01GM081293, Google, and the National Science Foundation un- der grant numbers IIS-0811562, CCF-1247088, IIS-1250956 and CCF-1414030. The views and conclusionscontained in this document are those of the author and should not be interpreted as representing the official
policies, either expressed or implied, of any sponsoring institution, the U.S. government or any other entity.
Keywords:grounded language learning, natural language processing, knowledge base construction, knowledgerepresentation, statisticallanguagemodeling, unsupervisedlearn- ing, semi supervised learning, bootstrapping, topic modeling, machine learning, proba- bilistic graphical models, ontology, grounding, information extraction.For Yair, with my love.
ivAbstract
This thesisis focused on buildingknowledge bases (KBs)for scientific do- mains. Specifically, we create structured representations of technical-domain information using unsupervised or semi-supervised learning methods. This work is inspired by recent advances in knowledge base construction based on Web text. However, in the technical domains we consider here, in addition to text corpora we have access to the objects named by text entities, as well as data associated with those objects. For example, in the software domain, we consider the implementation of classes in code repositories, and observe the way they are being used in programs. In the biomedical realm, biological on- tologies define interactions and relations between domain entities, and there is experimental information on entities such as proteins and genes. We consider the process ofgrounding, namely, linking entity mentions from text to exter- nal domain resources, including code repositories and biomedical ontologies, where objects can be uniquely identified. Grounding presents an opportunity for learning, not only how entities are discussed in text, but also what are their real-world properties. The main contribution of this thesis is in addressing challenges from the following research areas, in the context of learning about technical domains: (1)Knowledge representation:How should knowledge about technical do- mains be represented and used? (2)Grounding:How can existing resources of technical domains be used in learning? (3)Applications:What applications can benefit from structured knowledge bases dedicated to scientific data? We explore grounded learning and knowledge base construction for the biomedical and software domains. We first discuss approaches for improv- ing applications based on well-studied statistical language models. Next, we construct a deeper semantic representation of domain-entities by building a grounded ontology, where entities are linked to a code repository, and through an adaption of an ontology-driven KB learner to scientific input. Finally, we present a topic model framework for knowledge base construction, which jointly optimizes the KB schema and learned facts, and show that this frame- work produces high precision KBs in our two domains of interest. We discuss extensions to our model that allow: first, incorporating human input, leading to a semi-supervised learning process, and second, grounding the modeled entities with domain data. viAcknowledgments
The past 5 years at CMU have been a crazy and fun experience. I survived this journey thanks to the help and support of my friends and colleagues. Iamgratefultomyadvisor, WilliamCohen, fortransformingmefromaComputational Biologist to a Natural Language Processing researcher. His experience and knowledge, his patience, humor, and easy-going nature have all made our meetings both enjoyable and insightful. I especially appreciate his approach to research: starting with a few interesting examples and simple solutions, while keeping in mind the broad context of the problem and relation to other areas. I am fortunate to have in my thesis committee Tom Mitchell, Roni Rosenfeld and Alon Halevy. Tom has a vast knowledge of Artificial Intelligence and I appreciated getting his perspective on my work, always delivered with extraordinary kindness. It was a pleasure TAing for Roni, and learning from his interactive and engaging teaching style. I also had a fruitful and fun internship working in Alon"s group at Google, where I learned a lot about ontologies, attributes and coffee. My conversations with everyone in the committee, your advice, and support, have been valuable to me. My work was influenced by thoughts shared with the great individuals I had the op- portunity to meet, work with, and TA with, including: Bhavana Dalvi Mishra, Katie Ri- vard Mazaitis, William Yang Wang, Frank Lin, Ramnath Balasubramanyan, Tae Yano, Ni Lao, Nan Li, Mahesh Joshi, Einat Minkov, Malcolm Greaves, Freddy Chua, Premkumar Devanbu, Lin Tan, Song Wang, Partha Pratim Talukdar, Ndapa Nakashole, Estevam Hr- uschka, my internship hosts and co-workers: Steven Whang, Natasha Noy, Alon Halevy, Eric Sun, and my TA collaborators: Ariel Procaccia, Emma Brunskill, Danai Koutra, YairMovshovitz-Attias, Roni Rosenfeld and Ming Sun.
The surest way to make it through grad-school is with great friends. Thanks for the lunches, coffee breaks, parties, vacations, and for making it all so much fun! John Wright, Sarah Loos and Jeremy Karnowski, Gabe and Cat Weisz, Danai Koutra and Walter Lasecki, Aaditya Ramdas, Kate Taralova, Sam Gottlieb, Jo˜ao Martins, David Henriques,
vii Akshay Krishnamurthy, David Naylor, Galit Regev and Tzur Frenkel, Or Sheffet, Yuzi Nakamura, Mary Wootters, Jesse Dunietz, Nika Haghtalab, Deby Katz, Yu Zhao. Many thanks also to the administrative staff at CMU, who are always looking out for us. Thank you Deb Cavlovich, Catherine Copetas, Sharon Cavlovich and Sandy Winkler. I thank my parents Meir and Eti Atias, and my brothers Nir and Ben Atias, for their long-distance support, their fun visits to Pittsburgh, and for their encouragement through- out my studies. More than all, I thank my husband Yair Movshovitz-Attias, who has been here through it all. Together we have been through army service, undergrad and graduate studies. With your love, support, and mainly your endless stream of jokes, I know I can get anywhere. I am looking forward to more joint adventures. viiiContents
1 Introduction
11.1 Building Grounded Knowledge Bases
11.2 Thesis Statement
41.3 Thesis Roadmap
41.4 Chapter Synopsis
82 Statistical Language Modeling for a Software Domain Application
132.1 Introduction and Related Work
132.2 Method
142.2.1 Models
142.2.2 Testing Methodology
162.3 Experimental Settings
172.3.1 Data and Training Methodology
172.3.2 Evaluation
192.4 Results
202.5 Implementation and Corpus
242.6 Conclusions
243 Bootstrap Knowledge Base Learning for the Biomedical Domain
253.1 Introduction
263.2 Related Work
29ix
3.3 Implementation. . . . . . . . . . . . . . . . . . . . . . . . . . . . . . . 31
3.3.1 NELL"s Bootstrapping System
313.3.2 Text Corpora
313.3.3 Ontology
313.3.4 BioNELL"s Bootstrapping System
32PMI Collocation with the Category Name
32Rank-and-Learn Bootstrapping
333.4 Experimental Evaluation
333.4.1 Experimental Settings
33Configurations of the Algorithm
33Evaluation Methodology
34Data Sets
343.4.2 Extending Lexicons of Biomedical Categories
35Recovering a Closed Category Lexicon
35Extending Lexicons of Open Categories
383.4.3 Named-Entity Recognition using a Learned Lexicon
38Using a Complete Dictionary
39Using a Manually-Filtered Dictionary
40Using a Learned Lexicon
403.5 Conclusions
404 Grounded Software Ontology Construction using Coordinate Term Relation-
ships 434.1 Introduction
444.2 Related Work
454.2.1 Semantic Relation Discovery
454.2.2 Grounded Language Learning
484.2.3 Statistical Language Models for Software
484.3 Coordinate Term Discovery
48x
4.3.1 Baseline: Corpus Distributional Similarity. . . . . . . . . . . . . 49
4.3.2 Baseline: String Similarity
504.3.3 Entity Linking
504.3.4 Code Distributional Similarity
514.3.5 Code Hierarchies and Organization
514.4 Experimental Settings
534.4.1 Data Handling
534.4.2 Classification
544.5 Results
564.5.1 Classification and Feature Analysis
564.5.2 Evaluation by Manual Labeling
574.5.3 Taxonomy Construction
594.6 Conclusions
595 Topic Model Based Approach for Learning a Complete Knowledge Base
615.1 Introduction
625.2 KB-LDA
635.2.1 Inference in KB-LDA
675.2.2 Parallel Implementation
695.2.3 Data-driven discovery of topic concepts
695.3 Experimental Evaluation
705.3.1 Data
715.3.2 Evaluating the learned KB precision
71Precision of Instance Topics
71Precision of Topic Concepts
73Precision of Relations
74Precision of Hierarchy
765.3.3 Overlap of KB-LDA topics with human-provided labels
765.3.4 Extracting facts from an open IE resource
78xi
5.4 Related Work. . . . . . . . . . . . . . . . . . . . . . . . . . . . . . . . 79
5.5 Conclusions
806 Aligning Grounded and Learned Relations: A Comparison of Relations From
a Grounded Corpus with a Topic-Model Guided Knowledge Base 836.1 Introduction
846.2 Data
856.3 Methodology
866.4 Experimental results
886.4.1 Entity Analysis
896.4.2 Relation Analysis
916.4.3 Ontology Coherence
91Known versus Suggested Relations
92Comparison by Number of Topics
94Comparison with Ablated Models
95Full versus Sampled Corpus
966.4.4 Topic Coherence
976.4.5 Relation Coherence
996.5 Related Work
1016.6 Conclusions
1027 Conclusion
1037.1 Summary
1037.2 Closing Thoughts
1047.2.1 Limitations of Grounding
1047.2.2 Evaluating Knowledge Base Systems
1057.2.3 The Distinction Between Grounding and Semi-Supervision
1057.2.4 Latent Tensor Representation of Knowledge Bases
1067.3 Future Directions
106xii
7.3.1 Improving Software Language Modeling with a Software Ontology106
7.3.2 Learning a Grounded Ontology
1077.3.3 Semi-Supervised Ontology Learning
109Bibliography
111xiii xiv
List of Figures
1.1 Roadmap diagram. Each chapter in the thesis makes a contribution in
transforming input resources (e.g., a corpus, biomedical ontologies, or a code repository) for the task of building a knowledge base or addressing a domain specific application. In Chapter 2 we b uildmodels directly based on a code repository corpus in order to address a software domain task.Then, in Chapter
3 we use a higher le velreasoning of language, by b uild- ing a KB for the biomedical domain, based on biomedical ontologies. InChapter
4 we b uilda softw areontology from corpus, and in Chapter 5 we startfromacorpusandbuildacompleteKB.Finally, inChapter 6 wecom- pare relations from a learned KB, with relations originating in biomedical ontologies. For more details see Section 1.3 72.1 Resultsperproject, ontheaveragepercentageofcharacterssavedpercom-
ment, using n-gram (blue), LDA (light red) and Link-LDA (red) models trained on three training sets: IN (solid line), OUT (dashed line), and SO (dotted line). Top axis markf1,2,3g-grams, and bottom axis mark the number of topics used for LDA and Link-LDA models. The results are also summarized in Table 2.1 183.1 A sample from the BioCreative data set: (A) a list of gene identifiers (first
column) as well as alternative common names and symbols used to de- scribe each gene in the literature (second to last columns). The full data contains 7151 terms; and (B) sample abstract and two IDs of genes that have been annotated as being discussed in the text. In this example, the gene IDsFBgn0003204andFBgn0004034(can be found in the table) re- fer to theraspberryandyellowgenes which are mentioned in the abstract.The full data contains 108 abstracts.
28xv
3.2 PerformanceperlearningiterationforgenelexiconslearnedusingBioNELL
and NELL. 37(a) Precision 37
quotesdbs_dbs29.pdfusesText_35
[PDF] conclusion du premier voyage de christophe colomb
[PDF] lettre de christophe colomb 15 février 1493
[PDF] qu'est ce que l'altérité
[PDF] conclusion christophe colomb
[PDF] tp chromatographie sur couche mince des colorants alimentaires
[PDF] tp chromatographie 5ème
[PDF] protocole chromatographie m&m's
[PDF] tp chromatographie m&m's
[PDF] tp chromatographie colorants alimentaires
[PDF] chromatographie bonbon
[PDF] extraction colorant m&m's
[PDF] chromatographie m&m's college
[PDF] chromatographie 5ème exercice
[PDF] comment recuperer finalement la cafeine