[PDF] je cherche quelqu'un pour m'aider financièrement
[PDF] recherche aide a domicile personnes agées
[PDF] aide personne agée offre d'emploi
[PDF] tarif garde personne agée ? domicile
[PDF] y=ax+b graphique
[PDF] garde personne agée nuit particulier
[PDF] ménage chez personnes agées
[PDF] garde personne agee a son domicile
[PDF] cherche a garder personne agee a domicile
[PDF] calcul arithmétique de base
[PDF] ax2 bx c determiner a b et c
[PDF] opération arithmétique binaire
[PDF] rôle de la vitamine d dans l'organisme
[PDF] arithmétique synonyme
[PDF] role vitamine a
beamer-tu-logoLecture 11: Correlation and independence IfAis a constantmnmatrix, then 0 otherwise UW-Madison (Statistics)Stat 609 Lecture 112015 3 / 17 beamer-tu-logoThe marginal ofXisuniform(0;1), since for 0X(x) =Z For 0
[PDF] recherche aide a domicile personnes agées
[PDF] aide personne agée offre d'emploi
[PDF] tarif garde personne agée ? domicile
[PDF] y=ax+b graphique
[PDF] garde personne agée nuit particulier
[PDF] ménage chez personnes agées
[PDF] garde personne agee a son domicile
[PDF] cherche a garder personne agee a domicile
[PDF] calcul arithmétique de base
[PDF] ax2 bx c determiner a b et c
[PDF] opération arithmétique binaire
[PDF] rôle de la vitamine d dans l'organisme
[PDF] arithmétique synonyme
[PDF] role vitamine a
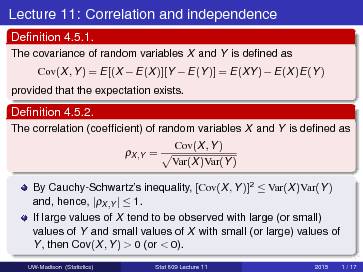
Definition 4.5.1.
The covariance of random variablesXandYis defined asCov(X;Y) =E[(XE(X)][YE(Y)] =E(XY)E(X)E(Y)
provided that the expectation exists.Definition 4.5.2. The correlation (coefficient) of random variablesXandYis defined as r X;Y=Cov(X;Y)pVar(X)Var(Y)By Cauchy-Schwartz"s inequality,[Cov(X;Y)]2Var(X)Var(Y) and, hence,jrX;Yj 1.If large values ofXtend to be observed with large (or small) values ofYand small values ofXwith small (or large) values of Y, then Cov(X;Y)>0 (or<0).UW-Madison (Statistics)Stat 609 Lecture 112015 1 / 17beamer-tu-logoIf Cov(X;Y) =0, then we say thatXandYare uncorrelated.The correlation is a standardized value of the covariance.
Theorem 4.5.6.
IfXandYare random variables andaandbare constants, then Var(aX+bY) =a2Var(X)+b2Var(Y)+2abCov(X;Y)Theorem 4.5.6 witha=b=1 implies that, ifXandYare positively correlated, then the variation inX+Yis greater than the sum of the variations inXandY; but if they are negatively correlated, then the variation inX+Yis less than the sum of the variations. This result is useful in statistical applications.Multivariate expectation The expectation of a random vectorX= (X1;:::;Xn)is defined as E(X) = (E(X1);:::;E(Xn)), provided thatE(Xi)exists for anyi. WhenMis a matrix whose(i;j)th element if a random variableXij, E(M)is defined as the matrix whose(i;j)th element isE(Xij), provided thatE(Xij)exists for any(i;j).UW-Madison (Statistics)Stat 609 Lecture 112015 2 / 17 beamer-tu-logoVariance-covariance matrix The concept of mean and variance can be extended to random vectors: for ann-dimensional random vectorX= (X1;:::;Xn), its mean isE(X)and its variance-covariance matrix isVar(X) =Ef[XE(X)][XE(X)]0g=E(XX0)E(X)E(X0)
which is annnsymmetric matrix whoseith diagonal element is the varianceVar(Xi)and(i;j)th off-diagonal element is the covarianceCov(Xi;Xj).
Var(X)is nonnegative definite.
If the rank ofVar(X)isrE(AX) =AE(X)andVar(AX) =AVar(X)A0Example 4.5.4.
The joint pdf of(X;Y)is
f(x;y) =1 0¥f(x;y)dy=Z
x+1 xdy=x+1x=1For 0 f Y(y) =Z
¥f(x;y)dx=Z
y 0dx=y0=y
and for 1y<2, f Y(y) =Z
¥f(x;y)dx=Z
1 y1dx=1(y1) =2y i.e., f Y(y) =8
:2y1y<2 y00 otherwise Thus,E(X) =1=2 and Var(X) =1=12, and
E(Y) =Z
1 0y2dy+Z
2 1y(2y)dy=13
+4113
(81) =1 Var(Y)=E(Y2)1=Z
1 0y3dy+Z
2 1y2(2y)dy1=14+1431541=16
UW-Madison (Statistics)Stat 609 Lecture 112015 4 / 17 beamer-tu-logoAlso, E(XY) =Z
1 0Z x+1 xxydydx=Z 1 0 x2+x2 dx=13 +14 =712 Hence,
Cov(X;Y) =E(XY)E(X)E(Y) =712
12 =112 r Theorem 4.5.7.
For random variablesXandY,jrX;Yj=1 iffP(Y=aX+b) =1 for constantsaandb, wherea>0 ifrX;Y=1 anda<0 ifrX;Y=1.The proof of this theorem is actually discussed when we study
Cauchy-Schwartz"s inequality (when the equality holds).If there is a line,y=ax+bwitha6=0, such that the values of the
2-dimensional random vector(X;Y)have a high probability of
being near this line, then the correlation betweenXandYwill be near 1 or1.UW-Madison (Statistics)Stat 609 Lecture 112015 5 / 17 beamer-tu-logoOn the other hand,XandYmay be highly related but have no linear relationship, and the correlation could be nearly 0.Example 4.5.8, 4.5.9. Consider(X;Y)having pdf
f(x;y) =10 00 otherwise This is the same as the pdf in Example 4.5.4 except that x0g. The same calculation as in Example 4.5.4 shows that r X;Y=p100=101, which is almost 1 (the first figure below). UW-Madison (Statistics)Stat 609 Lecture 112015 6 / 17 beamer-tu-logoConsider(X;Y)having a different pdf (the 2nd figure) f(x;y) =510 otherwise By symmetry,E(X) =0 andE(XY) =0; hence, Cov(X;Y) =rX;Y=0. There is actually a strong relationship betweenXandY. But this relationship is not linear.
quotesdbs_dbs2.pdfusesText_2
Y(y) =Z
¥f(x;y)dx=Z
y0dx=y0=y
and for 1y<2, fY(y) =Z
¥f(x;y)dx=Z
1 y1dx=1(y1) =2y i.e., fY(y) =8
:2y1y<2 y0Thus,E(X) =1=2 and Var(X) =1=12, and
E(Y) =Z
10y2dy+Z
21y(2y)dy=13
+4113(81) =1
Var(Y)=E(Y2)1=Z
10y3dy+Z
21y2(2y)dy1=14+1431541=16
UW-Madison (Statistics)Stat 609 Lecture 112015 4 / 17 beamer-tu-logoAlso,E(XY) =Z
1 0Z x+1 xxydydx=Z 1 0 x2+x2 dx=13 +14 =712Hence,
Cov(X;Y) =E(XY)E(X)E(Y) =712
12 =112 rTheorem 4.5.7.
For random variablesXandY,jrX;Yj=1 iffP(Y=aX+b) =1 forconstantsaandb, wherea>0 ifrX;Y=1 anda<0 ifrX;Y=1.The proof of this theorem is actually discussed when we study
Cauchy-Schwartz"s inequality (when the equality holds).If there is a line,y=ax+bwitha6=0, such that the values of the