Convex Optimization
This book is about convex optimization a special class of mathematical optimiza- tion problems
Optimisation convexe
convexe et des algorithmes plus spécifiquement des algorithmes proximaux de l'optimisation de fonctions convexes différentiables la seconde de l' ...
Eléments danalyse et doptimisation convexe.
Dans ce chapitre nous présentons quelques propriétés remarquables des fonctions convexes. Elle permettront de construire des algorithmes de minimisation dans
Optimisation et analyse convexe
OPTIMISATION. ET. ANALYSE CONVEXE. Exercices et problèmes corrigés avec rappels de cours. Jean-Baptiste Hiriart-Urruty. Collection dirigée par Daniel Guin.
Convex Optimization
4 sept. 2009 Boyd & Vandenberghe Convex Optimization
Convex Optimization – Boyd and Vandenberghe
surprisingly many problems can be solved via convex optimization. Introduction. 1–8 convex and y is a random variable with log-concave pdf then.
Optimisation des fonctions convexes
Optimisation des fonctions convexes D7 : Une fonction réelle f définie sur C convexe est dite convexe si pour tout (a
Convex Optimization Basics
Is this convex? What is the criterion function? The inequality and equality constraints? Feasible set? Is the solution unique when: • n
COURS OPTIMISATION Cours en Master M1 SITN Ionel Sorin
La fonction f est convexe (donc toute combinaison linéaire avec des coefficients stric- tement positifs de fonctions convexes est convexe). 2. Si au moins l'une
Non-Parametric Discrete Registration with Convex Optimisation
sition of similarity and regularisation term into two convex optimisation steps. This approach enables non-parametric registration with billions.
[PDF] Optimisation convexe - Institut de Mathématiques de Bordeaux
Ce document est un support pour le cours d'optimisation Il n'a donc pas vocation `a être complet mais vient en appui aux séances de cours
[PDF] Eléments danalyse et doptimisation convexe
19 fév 2020 · 4 1 Méthodes optimales en optimisation convexe différentiable 45 4 1 1 Cas convexe différentiable
[PDF] Cours en Master M1 SITN
2 2 1 Fonctions convexes strictement convexes fortement convexes 11 4 2 3 Applications de la théorie du point selle à l'optimisation
[PDF] Optimisation convexe : géométrie modélisation et applications
19 nov 2009 · 1 Géométrie et analyse convexe 2 Optimisation : idées exemples convexité 3 Optimisation de la production électrique en France
[PDF] Optimisation convexe non-lisse - ENS Lyon
In our opinion convex optimization is a natural next topic after advanced linear algebra and linear programming (Stephen Boyd and Lieven Vandenberghe)
[PDF] Optimisation linéaire & convexité
I 1 Introduction à la programmation linéaire 1 I 1 1 Un problème d'optimisation linéaire en dimension 2 II 2 4 Fonctions convexes
[PDF] Convexité en optimisation convexité forte
On dit que f est strictement convexe si l'inégalité ci-dessus est stricte pour x = y t ?]01[ Rappelons que toute fonction convexe possède une régularité
[PDF] Cours Apprentissage - ENS Math/Info Optimisation Convexe
16 oct 2015 · pdf pour la r`egle dite d'Armijo – Analyse théorique – Hypoth`eses pour résultat théorique simple : f deux fois dérivable convexe LI f (x)
[PDF] OPTIMISATION ET ANALYSE CONVEXE - Numilog
Pour les fonctions convexes différentiables on pourra consulter [12] Cha- pitre IV Section 4 par exemple * Exercice I 1 Soit f : Rn ?? R continûment
[PDF] compléments danalyse : convexité et optimisation - ENS Rennes
1 1 1 Généralités 1 1 2 Convexité dans les espaces de Hilbert 5 1 3 Convexité et convergence faible 12 2 Fonctions convexes
Comment montrer qu'un problème est convexe ?
Théorème 2.1 Un fonction f est convexe si et seulement si, pour tout (x, y) ? (dom(f))2 et ? ? 0 tels que y + ?(y ? x) ? dom(f), f satisfait : f(y + ?(y ? x)) ? f(y) + ?(f(y) ? f(x)).19 fév. 2020Comment la convexité permet d'optimiser certains marchés économiques ?
C'est un concept utile dans le cadre du trading sur obligations car la comparaison des durées des obligations peut permettre aux investisseurs d'anticiper le degré de variation du cours d'une obligation suite à un changement de taux d'intérêt.Comment montrer qu'une fonction est fortement convexe ?
Une fonction f : K ?? R est dite fortement convexe ou uniformément convexe ou ?-convexe ou ?-elliptique s'il existe ? > 0 tel que, pour tous (x, y) ? K2, t ? [0,1], f(tx + (1 ? t)y) ? tf(x) + (1 ? t)f(y) ? ? 2 t(1 ? t)x ? y2.- La fonction f est convexe sur I si sa dérivée f ' est croissante sur I, soit f ''(x) ? 0 pour tout x de I. La fonction f est concave sur I si sa dérivée f ' est décroissante sur I, soit f ''(x) ? 0 pour tout x de I. Soit la fonction f définie sur R par f (x) = 1 3 x3 ?9x2 + 4.
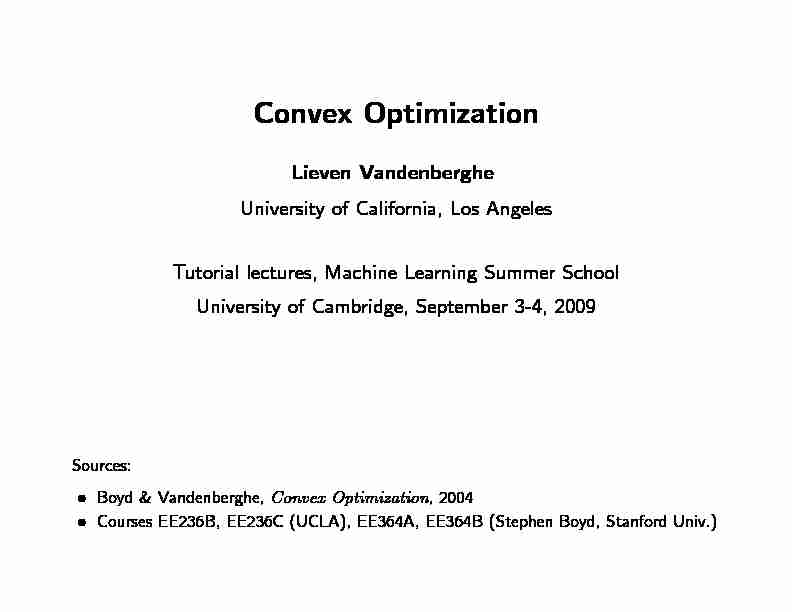
Convex Optimization
Lieven Vandenberghe
University of California, Los Angeles
Tutorial lectures, Machine Learning Summer School
University of Cambridge, September 3-4, 2009
Sources:
Boyd & Vandenberghe,Convex Optimization, 2004
Courses EE236B, EE236C (UCLA), EE364A, EE364B (Stephen Boyd, Stanford Univ.)Convex optimization - MLSS 2009
Introduction
mathematical optimization, modeling, complexityconvex optimization
recent history
1Mathematical optimization
minimizef0(x1,...,xn)x= (x1,x2,...,xn)are decision variables
f0(x1,x2,...,xn)gives the cost of choosingx
inequalities give constraints thatxmust satisfy a mathematical model of a decision, design, or estimation problemIntroduction2
Limits of mathematical optimization
how realistic is the model, and how certain are we about it? is the optimization problem tractable by existing numerical algorithms?Optimization research
modelinggeneric techniques for formulating tractable optimization problems algorithmsexpand class of problems that can be efficiently solvedIntroduction3
Complexity of nonlinear optimization
the general optimization problem is intractable even simple looking optimization problems can be very hardExamples
quadratic optimization problem with many constraintsminimizing a multivariate polynomial
Introduction4
The famous exception: Linear programming
minimizecTx=n? i=1c ixi widely used since Dantzig introduced the simplex algorithm in 1948 since 1950s, many applications in operations research, network optimization, finance, engineering,. . . extensive theory (optimality conditions, sensitivity, . . . ) there exist very efficient algorithms for solving linear programsIntroduction5
Solving linear programs
no closed-form expression for solution
widely available and reliable software
for some algorithms, can prove polynomial time problems with over105variables or constraints solved routinelyIntroduction6
Convex optimization problem
minimizef0(x) f includes least-squares problems and linear programs as special cases can be solved exactly, with similar complexity as LPs surprisingly many problems can be solved via convex optimizationIntroduction7
History
1940s: linear programming
minimizecTx1950s: quadratic programming
1960s: geometric programming
1990s: semidefinite programming, second-order cone programming, quadratically constrained quadratic programming, robust optimization, sum-of-squares programming, . . .Introduction8
New applications since 1990
linear matrix inequality techniques in controlcircuit design via geometric programming
support vector machine learning via quadratic programming semidefinite programming relaxations in combinatorial optimization ?1-norm optimization for sparse signal reconstruction applications in structural optimization, statistics, signal processing, communications, image processing, computer vision, quantum information theory, finance, . . .Introduction9
Algorithms
Interior-point methods
1984 (Karmarkar): first practical polynomial-time algorithm 1984-1990: efficient implementations for large-scale LPs around 1990 (Nesterov & Nemirovski): polynomial-time interior-point methods for nonlinear convex programming since 1990: extensions and high-quality software packagesFirst-order algorithms
similar to gradient descent, but with better convergence properties based on Nesterov"s 'optimal" methods from 1980s extend to certain nondifferentiable or constrained problemsIntroduction10
Outline
basic theory
-convex sets and functions -convex optimization problems -linear, quadratic, and geometric programmingcone linear programming and applications
-second-order cone programming -semidefinite programming some recent developments in algorithms (since 1990) -interior-point methods -fast gradient methods 10Convex optimization - MLSS 2009
Convex sets and functions
definition
basic examples and properties
operations that preserve convexity
11Convex set
contains line segment between any two points in the set xExamples: one convex, two nonconvex sets
Convex sets and functions12
Examples and properties
solution set of linear equationsAx=b(affine set) solution set of linear inequalitiesAx?b(polyhedron) set of positive semidefinite matricesSn+={X?Sn|X?0} image of a convex set under a linear transformation is convex inverse image of a convex set under a linear transformation is convexintersection of convex sets is convex
Convex sets and functions13
Example of intersection property
wherep(t) =x1cost+x2cos2t+···+xncosnt0π/32π/3π-101
t p(t) x1 x2 C -2-1012-2-1012 Cis intersection of infinitely many halfspaces, hence convexConvex sets and functions14
Convex function
domaindomfis a convex set and (x,f(x))(y,f(y)) fis concave if-fis convexConvex sets and functions15
Epigraph and sublevel set
a function is convex if and only its epigraph is a convex set epif f the sublevel sets of a convex function are convex (converse is false)Convex sets and functions16
Examples
expx,-logx,xlogxare convex
quadratic-over-linear functionxTx/tis convex inx,tfort >0 geometric mean(x1x2···xn)1/nis concave forx?0 logdetXis concave on set of positive definite matriceslog(ex1+···exn)is convex
linear and affine functions are convex and concavenorms are convex
Convex sets and functions17
Differentiable convex functions
differentiablefis convex if and only ifdomfis convex and f(y)≥f(x) +?f(x)T(y-x)for allx,y?domf (x,f(x))f(y) f(x) +?f(x)T(y-x) twice differentiablefis convex if and only ifdomfis convex and2f(x)?0for allx?domf
Convex sets and functions18
Operations that preserve convexity
methods for establishing convexity of a function1. verify definition
2. for twice differentiable functions, show?2f(x)?0
3. show thatfis obtained from simple convex functions by operations
that preserve convexitynonnegative weighted sum
composition with affine function
pointwise maximum and supremum
composition
minimization
perspective
Convex sets and functions19
Positive weighted sum&composition with affine function Nonnegative multiple:αfis convex iffis convex,α≥0 Sum:f1+f2convex iff1,f2convex (extends to infinite sums, integrals) Composition with affine function:f(Ax+b)is convex iffis convexExamples
log barrier for linear inequalities
f(x) =-m? i=1log(bi-aTix)(any) norm of affine function:f(x) =?Ax+b?
Convex sets and functions20
Pointwise maximum
f(x) = max{f1(x),...,fm(x)} is convex iff1, . . . ,fmare convexExample:sum ofrlargest components ofx?Rn
f(x) =x[1]+x[2]+···+x[r] is convex (x[i]isith largest component ofx) proof:Convex sets and functions21
Pointwise supremum
g(x) = sup y?Af(x,y) is convex iff(x,y)is convex inxfor eachy? AExample:maximum eigenvalue of symmetric matrix
max(X) = sup ?y?2=1yTXyConvex sets and functions22
Composition with scalar functions
composition ofg:Rn→Randh:R→R: f(x) =h(g(x)) fis convex if gconvex,hconvex and nondecreasing gconcave,hconvex and nonincreasing (if we assignh(x) =∞forx?domh)Examples
expg(x)is convex ifgis convex
1/g(x)is convex ifgis concave and positive
Convex sets and functions23
Vector composition
composition ofg:Rn→Rkandh:Rk→R: f(x) =h(g(x)) =h(g1(x),g2(x),...,gk(x)) fis convex if g iconvex,hconvex and nondecreasing in each argument g iconcave,hconvex and nonincreasing in each argument (if we assignh(x) =∞forx?domh)Examples
?mi=1loggi(x)is concave ifgiare concave and positivelog?mi=1expgi(x)is convex ifgiare convex
Convex sets and functions24
Minimization
g(x) = infy?Cf(x,y) is convex iff(x,y)is convex inx,yandCis a convex setExamples
distance to a convex setC:g(x) = infy?C?x-y?
optimal value of linear program as function of righthand side g(x) = infy:Ay?xcTy follows by taking f(x,y) =cTy,domf={(x,y)|Ay?x}Convex sets and functions25
Perspective
theperspectiveof a functionf:Rn→Ris the functiong:Rn×R→R, g(x,t) =tf(x/t) gis convex iffis convex ondomg={(x,t)|x/t?domf, t >0}Examples
perspective off(x) =xTxis quadratic-over-linear function g(x,t) =xTx t perspective of negative logarithmf(x) =-logxis relative entropy g(x,t) =tlogt-tlogxConvex sets and functions26
Convex optimization - MLSS 2009
Convex optimization problems
standard form
linear, quadratic, geometric programming
modeling languages
27Convex optimization problem
minimizef0(x) Ax=b f0,f1, . . . ,fmare convex functions
feasible set is convex
locally optimal points are globally optimal
tractable, both in theory and practice
Convex optimization problems28
Linear program (LP)
minimizecTx+d subject toGx?h Ax=binequality is componentwise vector inequality
convex problem with affine objective and constraint functionsfeasible set is a polyhedron
Px?-cConvex optimization problems29
Piecewise-linear minimization
minimizef(x) = maxi=1,...,m(aTix+bi) xa T ix+bif(x)Equivalent linear program
minimizet an LP with variablesx,t?RConvex optimization problems30
Linear discrimination
separate two sets of points{x1,...,xN},{y1,...,yM}by a hyperplane aTxi+b >0, i= 1,...,N
aTyi+b <0, i= 1,...,M
homogeneous ina,b, hence equivalent to the linear inequalities (ina,b) aConvex optimization problems31
Approximate linear separation of non-separable setsquotesdbs_dbs33.pdfusesText_39[PDF] modélisation et simulation d'un moteur ? courant continu matlab
[PDF] modélisation mcc
[PDF] simulation mcc simulink
[PDF] asservissement et regulation de vitesse d'un moteur a courant continu
[PDF] modélisation d'un moteur ? courant continu
[PDF] equation differentielle moteur courant continu
[PDF] schéma bloc moteur ? courant continu
[PDF] commande pid d'un moteur ? courant continu pdf
[PDF] modélisation machine asynchrone simulink
[PDF] onduleur triphasé matlab
[PDF] cours de modélisation financière sous excel
[PDF] modélisation financière pdf
[PDF] fiche de lecture les misérables victor hugo pdf
[PDF] modélisation financière exemple